I craft unique cereal names, stories, and ridiculously cute Cereal Baby images.
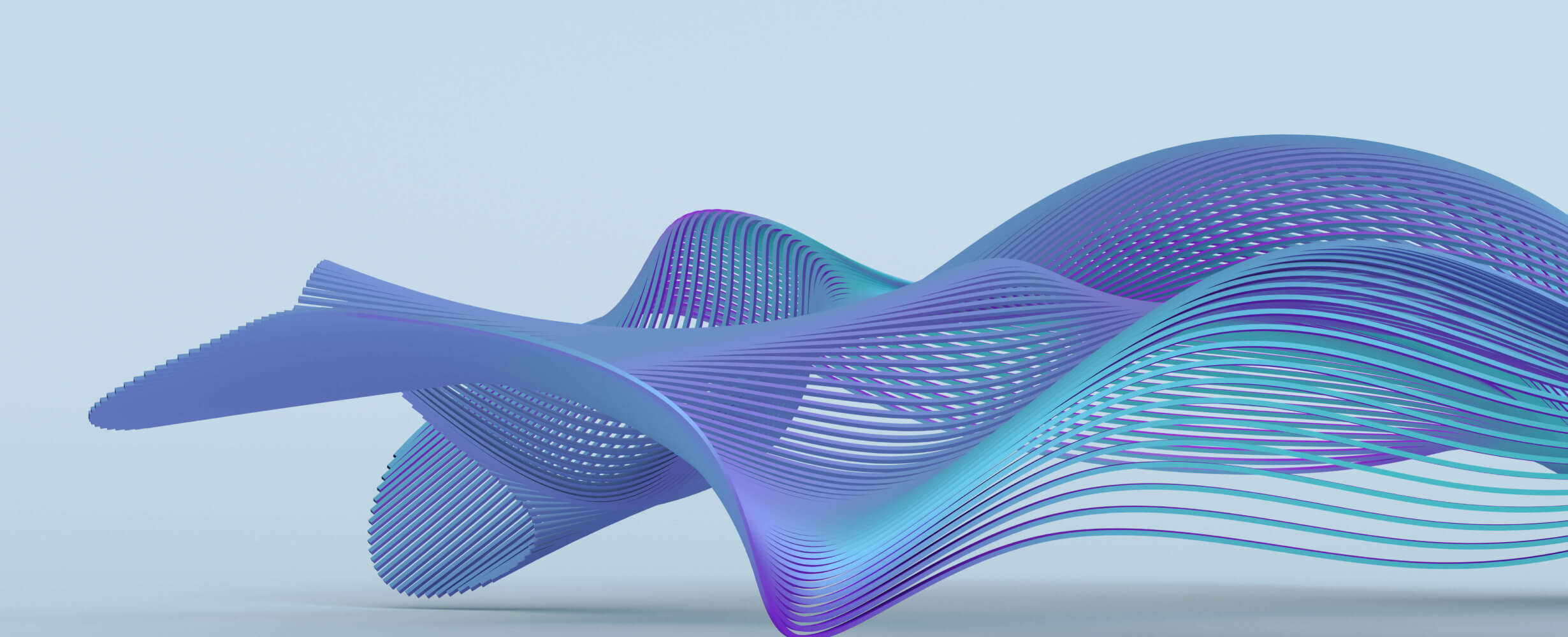
MCP-Server-Milvus
Milvus的模型上下文协议服务器
3 years
Works with Finder
1
Github Watches
1
Github Forks
6
Github Stars
MCP Server for Milvus
The Model Context Protocol (MCP) is an open protocol that enables seamless integration between LLM applications and external data sources and tools. Whether you're building an AI-powered IDE, enhancing a chat interface, or creating custom AI workflows, MCP provides a standardized way to connect LLMs with the context they need.
This repository contains a MCP server that provides access to Milvus vector database functionality.
Prerequisites
Before using this MCP server, ensure you have:
- Python 3.10 or higher
- A running Milvus instance (local or remote)
- uv installed (recommended for running the server)
Usage
The recommended way to use this MCP server is to run it directly with uv
without installation. This is how both Claude Desktop and Cursor are configured to use it in the examples below.
If you want to clone the repository:
git clone https://github.com/stephen37/mcp-server-milvus.git
cd mcp-server-milvus
Then you can run the server directly:
uv run src/mcp_server_milvus/server.py --milvus-uri http://localhost:19530
Supported Applications
This MCP server can be used with various LLM applications that support the Model Context Protocol:
- Claude Desktop: Anthropic's desktop application for Claude
- Cursor: AI-powered code editor with MCP support in its Composer feature
- Custom MCP clients: Any application implementing the MCP client specification
Usage with Claude Desktop
-
Install Claude Desktop from https://claude.ai/download
-
Open your Claude Desktop configuration:
- macOS:
~/Library/Application Support/Claude/claude_desktop_config.json
- macOS:
-
Add the following configuration:
{
"mcpServers": {
"milvus": {
"command": "/PATH/TO/uv",
"args": [
"--directory",
"/path/to/mcp-server-milvus/src/mcp_server_milvus",
"run",
"server.py",
"--milvus-uri",
"http://localhost:19530"
]
}
}
}
- Restart Claude Desktop
Usage with Cursor
Cursor also supports MCP tools through its Agent feature in Composer. You can add the Milvus MCP server to Cursor in two ways:
Option 1: Using Cursor Settings UI
-
Go to
Cursor Settings
>Features
>MCP
-
Click on the
+ Add New MCP Server
button -
Fill out the form:
-
Type: Select
stdio
(since you're running a command) -
Name:
milvus
-
Command:
/PATH/TO/uv --directory /path/to/mcp-server-milvus/src/mcp_server_milvus run server.py --milvus-uri http://127.0.0.1:19530
⚠️ Note: Use
127.0.0.1
instead oflocalhost
to avoid potential DNS resolution issues. -
Type: Select
Option 2: Using Project-specific Configuration (Recommended)
Create a .cursor/mcp.json
file in your project root:
-
Create the
.cursor
directory in your project root:mkdir -p /path/to/your/project/.cursor
-
Create a
mcp.json
file with the following content:{ "mcpServers": { "milvus": { "command": "/PATH/TO/uv", "args": [ "--directory", "/path/to/mcp-server-milvus/src/mcp_server_milvus", "run", "server.py", "--milvus-uri", "http://127.0.0.1:19530" ] } } }
-
Restart Cursor or reload the window
After adding the server, you may need to press the refresh button in the MCP settings to populate the tool list. The Composer Agent will automatically use the Milvus tools when relevant to your queries.
Verifying the Integration
To verify that Cursor has successfully integrated with your Milvus MCP server:
- Open Cursor Settings > Features > MCP
- Check that "Milvus" appears in the list of MCP servers
- Verify that the tools are listed (e.g., milvus_list_collections, milvus_vector_search, etc.)
- If the server is enabled but shows an error, check the Troubleshooting section below
Available Tools
The server provides the following tools:
Search and Query Operations
-
milvus-text-search
: Search for documents using full text search- Parameters:
-
collection_name
: Name of collection to search -
query_text
: Text to search for -
limit
: Maximum results (default: 5) -
output_fields
: Fields to include in results -
drop_ratio
: Proportion of low-frequency terms to ignore (0.0-1.0)
-
- Parameters:
-
milvus-vector-search
: Perform vector similarity search on a collection- Parameters:
-
collection_name
: Name of collection to search -
vector
: Query vector -
vector_field
: Field containing vectors to search (default: "vector") -
limit
: Maximum results (default: 5) -
output_fields
: Fields to include in results -
metric_type
: Distance metric (COSINE, L2, IP) (default: "COSINE") -
filter_expr
: Optional filter expression
-
- Parameters:
-
milvus-hybrid-search
: Perform hybrid search combining vector similarity and attribute filtering- Parameters:
-
collection_name
: Name of collection to search -
vector
: Query vector -
vector_field
: Field containing vectors to search (default: "vector") -
filter_expr
: Filter expression for metadata -
limit
: Maximum results (default: 5) -
output_fields
: Fields to include in results -
metric_type
: Distance metric (COSINE, L2, IP) (default: "COSINE")
-
- Parameters:
-
milvus-multi-vector-search
: Perform vector similarity search with multiple query vectors- Parameters:
-
collection_name
: Name of collection to search -
vectors
: List of query vectors -
vector_field
: Field containing vectors to search (default: "vector") -
limit
: Maximum results per query (default: 5) -
output_fields
: Fields to include in results -
metric_type
: Distance metric (COSINE, L2, IP) (default: "COSINE") -
filter_expr
: Optional filter expression
-
- Parameters:
-
milvus-query
: Query collection using filter expressions- Parameters:
-
collection_name
: Name of collection to query -
filter_expr
: Filter expression (e.g. 'age > 20') -
output_fields
: Fields to include in results -
limit
: Maximum results (default: 10)
-
- Parameters:
-
milvus-count
: Count entities in a collection- Parameters:
-
collection_name
: Name of the collection -
filter_expr
: Optional filter expression
-
- Parameters:
Collection Management
-
milvus-list-collections
: List all collections in the database -
milvus-collection-info
: Get detailed information about a collection- Parameters:
-
collection_name
: Name of the collection
-
- Parameters:
-
milvus-get-collection-stats
: Get statistics about a collection- Parameters:
-
collection_name
: Name of collection
-
- Parameters:
-
milvus-create-collection
: Create a new collection with specified schema- Parameters:
-
collection_name
: Name for the new collection -
schema
: Collection schema definition -
index_params
: Optional index parameters
-
- Parameters:
-
milvus-load-collection
: Load a collection into memory for search and query- Parameters:
-
collection_name
: Name of collection to load -
replica_number
: Number of replicas (default: 1)
-
- Parameters:
-
milvus-release-collection
: Release a collection from memory- Parameters:
-
collection_name
: Name of collection to release
-
- Parameters:
-
milvus-get-query-segment-info
: Get information about query segments- Parameters:
-
collection_name
: Name of collection
-
- Parameters:
-
milvus-get-collection-loading-progress
: Get the loading progress of a collection- Parameters:
-
collection_name
: Name of collection
-
- Parameters:
Data Operations
-
milvus-insert-data
: Insert data into a collection- Parameters:
-
collection_name
: Name of collection -
data
: Dictionary mapping field names to lists of values
-
- Parameters:
-
milvus-bulk-insert
: Insert data in batches for better performance- Parameters:
-
collection_name
: Name of collection -
data
: Dictionary mapping field names to lists of values -
batch_size
: Number of records per batch (default: 1000)
-
- Parameters:
-
milvus-upsert-data
: Upsert data into a collection (insert or update if exists)- Parameters:
-
collection_name
: Name of collection -
data
: Dictionary mapping field names to lists of values
-
- Parameters:
-
milvus-delete-entities
: Delete entities from a collection based on filter expression- Parameters:
-
collection_name
: Name of collection -
filter_expr
: Filter expression to select entities to delete
-
- Parameters:
-
milvus-create-dynamic-field
: Add a dynamic field to an existing collection- Parameters:
-
collection_name
: Name of collection -
field_name
: Name of the new field -
data_type
: Data type of the field -
description
: Optional description
-
- Parameters:
Index Management
-
milvus-create-index
: Create an index on a vector field- Parameters:
-
collection_name
: Name of collection -
field_name
: Field to index -
index_type
: Type of index (IVF_FLAT, HNSW, etc.) (default: "IVF_FLAT") -
metric_type
: Distance metric (COSINE, L2, IP) (default: "COSINE") -
params
: Additional index parameters
-
- Parameters:
-
milvus-get-index-info
: Get information about indexes in a collection- Parameters:
-
collection_name
: Name of collection -
field_name
: Optional specific field to get index info for
-
- Parameters:
Environment Variables
-
MILVUS_URI
: Milvus server URI (can be set instead of --milvus-uri) -
MILVUS_TOKEN
: Optional authentication token -
MILVUS_DB
: Database name (defaults to "default")
Development
To run the server directly:
uv run server.py --milvus-uri http://localhost:19530
Examples
Using Claude Desktop
Example 1: Listing Collections
What are the collections I have in my Milvus DB?
Claude will then use MCP to check this information on our Milvus DB.
I'll check what collections are available in your Milvus database.
> View result from milvus-list-collections from milvus (local)
Here are the collections in your Milvus database:
1. rag_demo
2. test
3. chat_messages
4. text_collection
5. image_collection
6. customized_setup
7. streaming_rag_demo
Example 2: Searching for Documents
Find documents in my text_collection that mention "machine learning"
Claude will use the full-text search capabilities of Milvus to find relevant documents:
I'll search for documents about machine learning in your text_collection.
> View result from milvus-text-search from milvus (local)
Here are the documents I found that mention machine learning:
[Results will appear here based on your actual data]
Using Cursor
Example: Creating a Collection
In Cursor's Composer, you can ask:
Create a new collection called 'articles' in Milvus with fields for title (string), content (string), and a vector field (128 dimensions)
Cursor will use the MCP server to execute this operation:
I'll create a new collection called 'articles' with the specified fields.
> View result from milvus-create-collection from milvus (local)
Collection 'articles' has been created successfully with the following schema:
- title: string
- content: string
- vector: float vector[128]
Troubleshooting
Common Issues
Connection Errors
If you see errors like "Failed to connect to Milvus server":
- Verify your Milvus instance is running:
docker ps
(if using Docker) - Check the URI is correct in your configuration
- Ensure there are no firewall rules blocking the connection
- Try using
127.0.0.1
instead oflocalhost
in the URI
Authentication Issues
If you see authentication errors:
- Verify your
MILVUS_TOKEN
is correct - Check if your Milvus instance requires authentication
- Ensure you have the correct permissions for the operations you're trying to perform
Tool Not Found
If the MCP tools don't appear in Claude Desktop or Cursor:
- Restart the application
- Check the server logs for any errors
- Verify the MCP server is running correctly
- Press the refresh button in the MCP settings (for Cursor)
Getting Help
If you continue to experience issues:
- Check the GitHub Issues for similar problems
- Join the Zilliz Community Discord for support
- File a new issue with detailed information about your problem
相关推荐
Confidential guide on numerology and astrology, based of GG33 Public information
A geek-themed horoscope generator blending Bitcoin prices, tech jargon, and astrological whimsy.
Therapist adept at identifying core issues and offering practical advice with images.
A world class elite tech co-founder entrepreneur, expert in software development, entrepreneurship, marketing, coaching style leadership and aligned with ambition for excellence, global market penetration and worldy perspectives.
A medical specialist offering assistance grounded in clinical guidelines. Disclaimer: This is intended for research and is NOT safe for clinical use!
Reviews
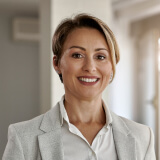
user_gV3fgSjP
I've been using Remote MCP Server on Cloudflare by ilamanov, and it's been a game-changer for my projects. The setup is straightforward, the server performance is exceptional, and Cloudflare integration ensures top-notch security and speed. If you're looking for a reliable remote server solution, I highly recommend checking it out.