I craft unique cereal names, stories, and ridiculously cute Cereal Baby images.
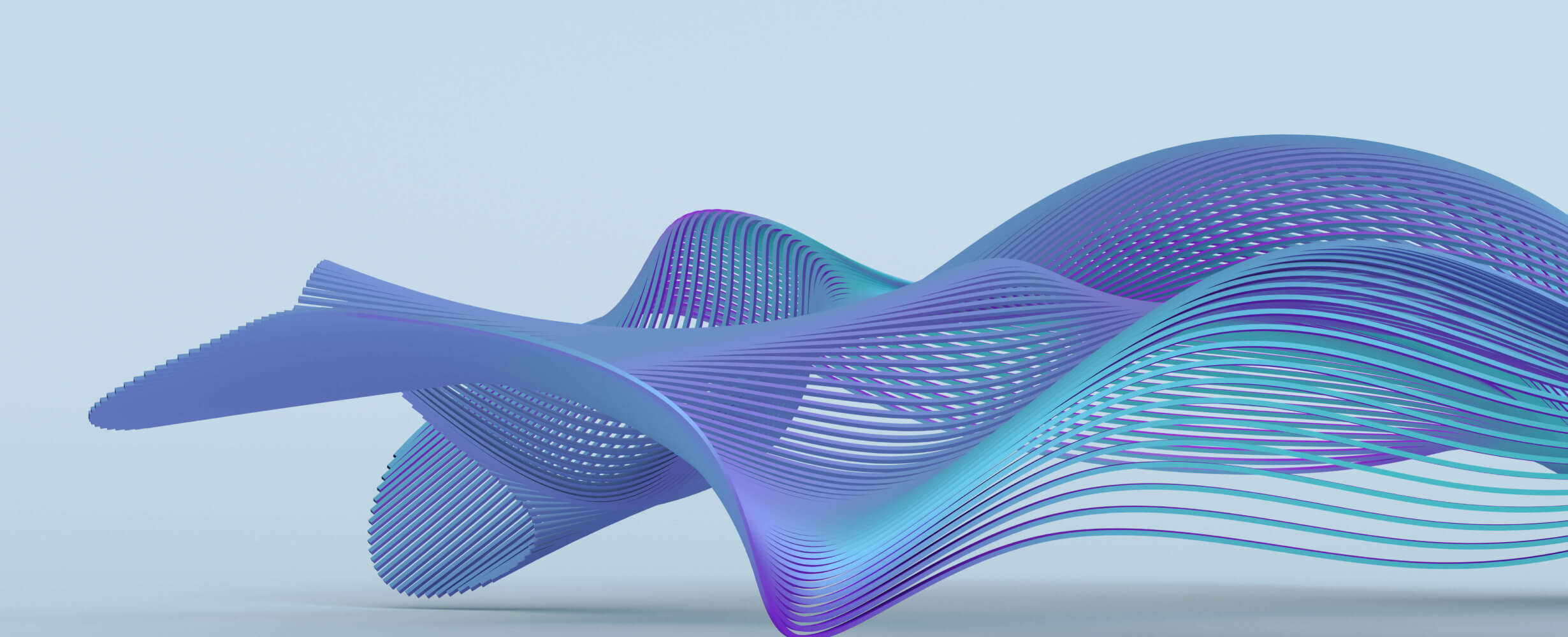
Databricks-MCP-Server
用于与Databricks服务交互的模型完成协议(MCP)服务器
1
Github Watches
7
Github Forks
11
Github Stars
Databricks MCP Server
A Model Completion Protocol (MCP) server for Databricks that provides access to Databricks functionality via the MCP protocol. This allows LLM-powered tools to interact with Databricks clusters, jobs, notebooks, and more.
Features
- MCP Protocol Support: Implements the MCP protocol to allow LLMs to interact with Databricks
- Databricks API Integration: Provides access to Databricks REST API functionality
- Tool Registration: Exposes Databricks functionality as MCP tools
- Async Support: Built with asyncio for efficient operation
Available Tools
The Databricks MCP Server exposes the following tools:
- list_clusters: List all Databricks clusters
- create_cluster: Create a new Databricks cluster
- terminate_cluster: Terminate a Databricks cluster
- get_cluster: Get information about a specific Databricks cluster
- start_cluster: Start a terminated Databricks cluster
- list_jobs: List all Databricks jobs
- run_job: Run a Databricks job
- list_notebooks: List notebooks in a workspace directory
- export_notebook: Export a notebook from the workspace
- list_files: List files and directories in a DBFS path
- execute_sql: Execute a SQL statement
Installation
Prerequisites
- Python 3.10 or higher
-
uv
package manager (recommended for MCP servers)
Setup
-
Install
uv
if you don't have it already:# MacOS/Linux curl -LsSf https://astral.sh/uv/install.sh | sh # Windows (in PowerShell) irm https://astral.sh/uv/install.ps1 | iex
Restart your terminal after installation.
-
Clone the repository:
git clone https://github.com/JustTryAI/databricks-mcp-server.git cd databricks-mcp-server
-
Set up the project with
uv
:# Create and activate virtual environment uv venv # On Windows .\.venv\Scripts\activate # On Linux/Mac source .venv/bin/activate # Install dependencies in development mode uv pip install -e . # Install development dependencies uv pip install -e ".[dev]"
-
Set up environment variables:
# Windows set DATABRICKS_HOST=https://your-databricks-instance.azuredatabricks.net set DATABRICKS_TOKEN=your-personal-access-token # Linux/Mac export DATABRICKS_HOST=https://your-databricks-instance.azuredatabricks.net export DATABRICKS_TOKEN=your-personal-access-token
You can also create an
.env
file based on the.env.example
template.
Running the MCP Server
To start the MCP server, run:
# Windows
.\start_mcp_server.ps1
# Linux/Mac
./start_mcp_server.sh
These wrapper scripts will execute the actual server scripts located in the scripts
directory. The server will start and be ready to accept MCP protocol connections.
You can also directly run the server scripts from the scripts directory:
# Windows
.\scripts\start_mcp_server.ps1
# Linux/Mac
./scripts/start_mcp_server.sh
Querying Databricks Resources
The repository includes utility scripts to quickly view Databricks resources:
# View all clusters
uv run scripts/show_clusters.py
# View all notebooks
uv run scripts/show_notebooks.py
Project Structure
databricks-mcp-server/
├── src/ # Source code
│ ├── __init__.py # Makes src a package
│ ├── __main__.py # Main entry point for the package
│ ├── main.py # Entry point for the MCP server
│ ├── api/ # Databricks API clients
│ ├── core/ # Core functionality
│ ├── server/ # Server implementation
│ │ ├── databricks_mcp_server.py # Main MCP server
│ │ └── app.py # FastAPI app for tests
│ └── cli/ # Command-line interface
├── tests/ # Test directory
├── scripts/ # Helper scripts
│ ├── start_mcp_server.ps1 # Server startup script (Windows)
│ ├── run_tests.ps1 # Test runner script
│ ├── show_clusters.py # Script to show clusters
│ └── show_notebooks.py # Script to show notebooks
├── examples/ # Example usage
├── docs/ # Documentation
└── pyproject.toml # Project configuration
See project_structure.md
for a more detailed view of the project structure.
Development
Code Standards
- Python code follows PEP 8 style guide with a maximum line length of 100 characters
- Use 4 spaces for indentation (no tabs)
- Use double quotes for strings
- All classes, methods, and functions should have Google-style docstrings
- Type hints are required for all code except tests
Linting
The project uses the following linting tools:
# Run all linters
uv run pylint src/ tests/
uv run flake8 src/ tests/
uv run mypy src/
Testing
The project uses pytest for testing. To run the tests:
# Run all tests with our convenient script
.\scripts\run_tests.ps1
# Run with coverage report
.\scripts\run_tests.ps1 -Coverage
# Run specific tests with verbose output
.\scripts\run_tests.ps1 -Verbose -Coverage tests/test_clusters.py
You can also run the tests directly with pytest:
# Run all tests
uv run pytest tests/
# Run with coverage report
uv run pytest --cov=src tests/ --cov-report=term-missing
A minimum code coverage of 80% is the goal for the project.
Documentation
- API documentation is generated using Sphinx and can be found in the
docs/api
directory - All code includes Google-style docstrings
- See the
examples/
directory for usage examples
Examples
Check the examples/
directory for usage examples. To run examples:
# Run example scripts with uv
uv run examples/direct_usage.py
uv run examples/mcp_client_usage.py
Contributing
Contributions are welcome! Please feel free to submit a Pull Request.
- Ensure your code follows the project's coding standards
- Add tests for any new functionality
- Update documentation as necessary
- Verify all tests pass before submitting
License
This project is licensed under the MIT License - see the LICENSE file for details.
相关推荐
Evaluator for marketplace product descriptions, checks for relevancy and keyword stuffing.
Emulating Dr. Jordan B. Peterson's style in providing life advice and insights.
Confidential guide on numerology and astrology, based of GG33 Public information
Your go-to expert in the Rust ecosystem, specializing in precise code interpretation, up-to-date crate version checking, and in-depth source code analysis. I offer accurate, context-aware insights for all your Rust programming questions.
Reviews
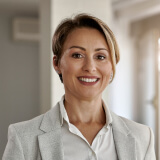
user_erSQ0CvD
I'm thoroughly impressed with the databricks-mcp-server by JustTryAI. It's an extremely efficient and reliable server that enhances the Databricks experience significantly. The integration is seamless and the performance boost is noticeable. Highly recommend this for anyone looking to optimize their Databricks operations! Check it out: https://github.com/JustTryAI/databricks-mcp-server