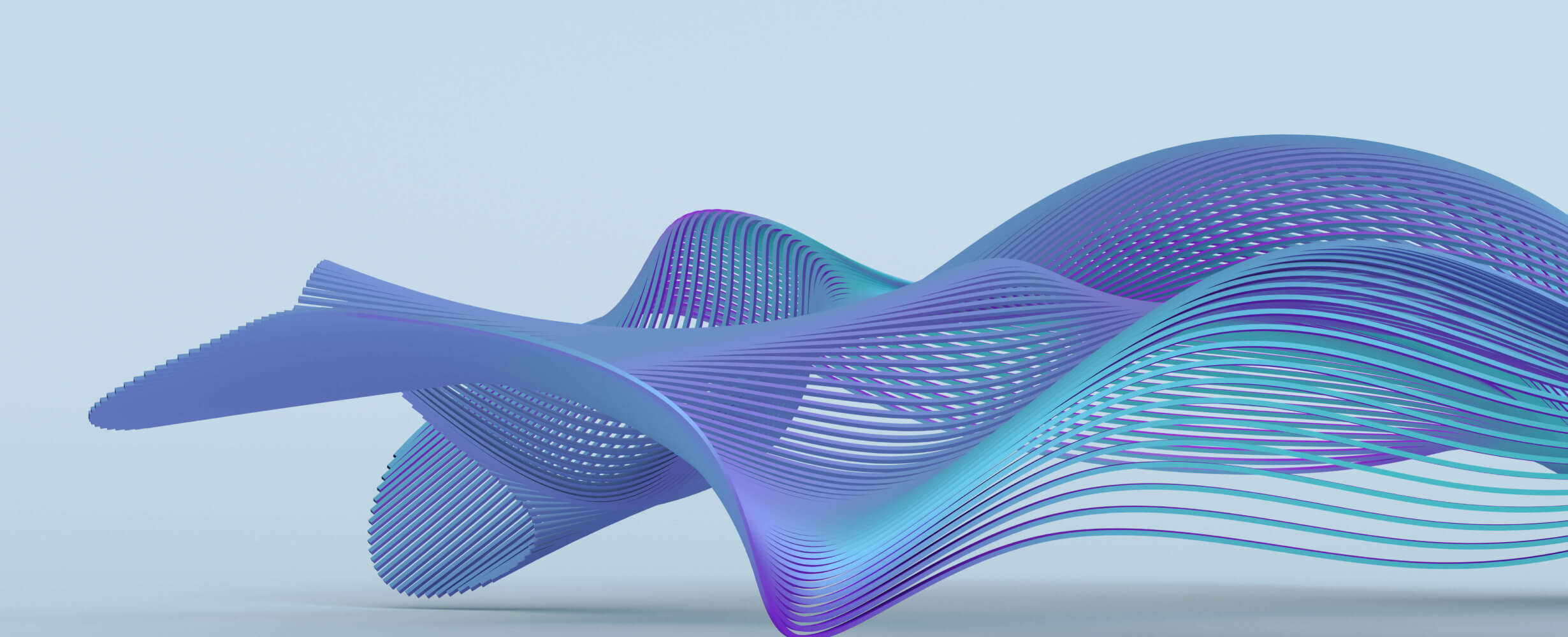
Jupyter-Notebook-MCP
Jupyter笔记本的模型上下文协议(MCP)
2
Github Watches
3
Github Forks
22
Github Stars
JupyterMCP - Jupyter Notebook Model Context Protocol Integration
JupyterMCP connects Jupyter Notebook to Claude AI through the Model Context Protocol (MCP), allowing Claude to directly interact with and control Jupyter Notebooks. This integration enables AI-assisted code execution, data analysis, visualization, and more.
⚠️ Compatibility Warning
This tool is compatible ONLY with Jupyter Notebook version 6.x.
It does NOT work with:
- Jupyter Lab
- Jupyter Notebook v7.x
- VS Code Notebooks
- Google Colab
- Any other notebook interfaces
Features
- Two-way communication: Connect Claude AI to Jupyter Notebook through a WebSocket-based server
- Cell manipulation: Insert, execute, and manage notebook cells
- Notebook management: Save notebooks and retrieve notebook information
- Cell execution: Run specific cells or execute all cells in a notebook
- Output retrieval: Get output content from executed cells with text limitation options
Components
The system consists of three main components:
-
WebSocket Server (
jupyter_ws_server.py
): Sets up a WebSocket server inside Jupyter that bridges communication between notebook and external clients -
Client JavaScript (
client.js
): Runs in the notebook to handle operations (inserting cells, executing code, etc.) -
MCP Server (
jupyter_mcp_server.py
): Implements the Model Context Protocol and connects to the WebSocket server
Installation
Prerequisites
- Python 3.12 or newer (probably also work with older versions, but not tested)
-
uv
package manager - Claude AI desktop application
Installing uv
If you're on Mac:
brew install uv
On Windows (PowerShell):
powershell -ExecutionPolicy ByPass -c "irm https://astral.sh/uv/install.ps1 | iex"
For other platforms, see the uv installation guide.
Setup
-
Clone or download this repository to your computer:
git clone https://github.com/jjsantos01/jupyter-notebook-mcp.git
-
Create virtual environment with required packages an install
jupyter-mcp
kernel, so it can be recognized by your jupyter installation, if you had one before.uv run python -m ipykernel install --name jupyter-mcp
-
(optional) Install additional Python packages for your analysis:
uv pip install seaborn
-
Configure Claude desktop integration: Go to
Claude
>Settings
>Developer
>Edit Config
>claude_desktop_config.json
to include the following:{ "mcpServers": { "jupyter": { "command": "uv", "args": [ "--directory", "/ABSOLUTE/PATH/TO/PARENT/REPO/FOLDER/src", "run", "jupyter_mcp_server.py" ] } } }
Replace
/ABSOLUTE/PATH/TO/
with the actual path to thesrc
folder on your system. For example:- Windows:
"C:\\Users\\MyUser\\GitHub\\jupyter-notebook-mcp\\src\\"
- Mac:
/Users/MyUser/GitHub/jupyter-notebook-mcp/src/
If you had previously opened Claude, then
File
>Exit
and open it again. - Windows:
Usage
Starting the Connection
-
Start your Jupyter Notebook (version 6.x) server:
uv run jupyter nbclassic
-
Create a new Jupyter Notebook and make sure that you choose the
jupyter-mcp
kernel:kernel
->change kernel
->jupyter-mcp
-
In a notebook cell, run the following code to initialize the WebSocket server:
import sys sys.path.append('/path/to/jupyter-notebook-mcp/src') # Add the path to where the scripts are located from jupyter_ws_server import setup_jupyter_mcp_integration # Start the WebSocket server inside Jupyter server, port = setup_jupyter_mcp_integration()
Don't forget to replace here
'/path/to/jupyter-notebook-mcp/src'
withsrc
folder on your system. For example:- Windows:
"C:\\Users\\MyUser\\GitHub\\jupyter-notebook-mcp\\src\\"
- Mac:
/Users/MyUser/GitHub/jupyter-notebook-mcp/src/
- Windows:
-
Launch Claude desktop with MCP enabled.
Using with Claude
Once connected, Claude will have access to the following tools:
-
ping
- Check server connectivity -
insert_and_execute_cell
- Insert a cell at the specified position and execute it -
save_notebook
- Save the current Jupyter notebook -
get_cells_info
- Get information about all cells in the notebook -
get_notebook_info
- Get information about the current notebook -
run_cell
- Run a specific cell by its index -
run_all_cells
- Run all cells in the notebook -
get_cell_text_output
- Get the output content of a specific cell -
get_image_output
- Get the images output of a specific cell -
edit_cell_content
- Edit the content of an existing cell -
set_slideshow_type
- Set the slide show type for cell
⚠️ DISCLAIMER
This is an experimental project and should be used with caution. This tool runs arbitrary Python code in your computer, which could potentially modify or delete data if not used carefully. Always back up your important projects and data.
Example Prompts
Ask Claude to perform notebook operations:
Python example
You can check the example notebook and the video demo
You have access to a Jupyter Notebook server.
I need to create a presentation about Python's Seaborn library.
The content is as follows:
- What is Seaborn?
- Long vs. Wide data format
- Advantages of Seaborn over Matplotlib
- Commonly used Seaborn functions
- Live demonstration (comparison of Seaborn vs. Matplotlib)
- Bar plot
- Line plot
- Scatter plot
For each concept, I want the main explanations provided in markdown cells, followed by one or more Python code cells demonstrating its usage. Keep the text concise—the cells shouldn't exceed 10 lines each.
Use appropriate slideshow types for each cell to make the presentation visually appealing.
Check Here the full conversation
Stata example
For this example, you need the Stata Software (v17 or later), which is not open source. If you already have Stata, you need to install the stata-setup
package:
uv pip install stata-setup
Then, at the begining of your notebook, you need to additionally include:
import stata_setup
stata_setup.config('your_stata_installation_directory', 'your_stata_edition')
You can check the example notebook and the video demo
This exercise comes from Professor John Robert Warren webpage
You have access to a Jupyter Notebook server. By default it runs Python, but you can run Stata (v18) code in this server using the %%stata magic, for example:
%%stata
display "hello world"
Run the available tools to solve the exercise, execute the code, and interpret the results.
**EXERCISE:**
In this exercise, you will use data from the American Community Survey (ACS). The ACS is a product of the U.S. Census Bureau and involves interviewing millions of Americans each year. For an introduction to the ACS, visit the ACS website (here).
For this exercise, I have created a data file containing two variables collected from respondents of the 2010 ACS who lived in one of two metropolitan areas: Minneapolis/St Paul and Duluth/Superior. The two variables are: (1) People's poverty status and (2) the time it takes people to commute to work.
Use STATA syntax files you already have (from the first assignment or class examples) and modify them to accomplish the following goals.
1. Read the data file (`"./stata_assignment_2.dat"`) for this assignment into STATA.
2. Be sure to declare "zero" as a missing value for `TRANTIME`, the commuting time variable.
3. Create a new dichotomous poverty variable that equals "1" if a person's income-to-poverty-line ratio (`POVRATIO`) is less than 100, and "0" otherwise; see the bottom of the assignment for an example of how to do this in STATA.
4. Separately for Minneapolis/St Paul and Duluth/Superior, produce:
- a histogram of the commuting time (`TRANTIME`) variable.
- measures of central tendency and spread for commuting time.
- a frequency distribution for the poverty status (0 vs 1) variable.
5. Separately for Minneapolis/St Paul and Duluth/Superior, use STATA code to produce:
- a 95% confidence interval for the mean commuting time.
- a 95% confidence interval for the proportion of people who are poor. See below for an example of how to do this in STATA.
Use the results from step #4 above to:
6. Separately for Minneapolis/St Paul and Duluth/Superior, manually calculate:
- a 95% confidence interval for the mean commuting time.
- a 95% confidence interval for the proportion of people who are poor.
7. Confirm that your answers from steps #5 and #6 match.
Based on the results above, answer this question:
8. How do you interpret the confidence intervals calculated in steps #5 and #6 above?
9. Finally, create a do file (.do) with the all the Stata code and the answers as comments.
---
**DESCRIPTION OF VARIABLES IN "STATA ASSIGNMENT 2.DAT"**
**METAREAD** (Column 4-7)
Metropolitan Area
- `2240`: Duluth-Superior, MN/WI
- `5120`: Minneapolis-St. Paul, MN
**POVRATIO** (Column 18-20)
Ratio of person's income to the poverty threshold:
- `<100`: Below Poverty Line
- `100`: At Poverty Line
- `>100`: Above Poverty Line
**TRANTIME** (Column 21-23)
Travel time to work
- `0`: Zero minutes
- `1`: 1 Minute
- etc.
Check Here the full conversation
Testing with External Client
You can test the functionality without using Claude Desktop with the included external client:
uv run python src/jupyter_ws_external_client.py
This will provide an interactive menu to test some available functions.
For automated testing of all commands:
uv run python src/jupyter_ws_external_client.py --batch
Troubleshooting
- Connection Issues: If you experience connection timeouts, the client includes a reconnection mechanism. You can also try restarting the WebSocket server.
- Cell Execution Problems: If cell execution doesn't work, check that the cell content is valid Python/Markdown and that the notebook kernel is running.
- WebSocket Port Conflicts: If the default port (8765) is already in use, the server will automatically try to find an available port.
Limitations
- Only supports Jupyter Notebook 6.x
- Text output from cells is limited to 1500 characters by default
- Does not support advanced Jupyter widget interactions
- Connection may timeout after periods of inactivity
License
Other Jupyter MCPs
This project is inspired by similar MCP integrations for Jupyter as:
相关推荐
Confidential guide on numerology and astrology, based of GG33 Public information
Take an adjectivised noun, and create images making it progressively more adjective!
Embark on a thrilling diplomatic quest across a galaxy on the brink of war. Navigate complex politics and alien cultures to forge peace and avert catastrophe in this immersive interstellar adventure.
Reviews
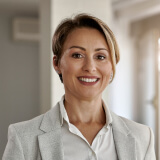
user_UhubcWYl
As a dedicated user of jupyter-notebook-mcp, I can confidently say this tool enhances the standard Jupyter Notebook experience. Developed by jjsantos01, it offers seamless integration and much-needed functionality. The user-friendly interface and comprehensive documentation make it accessible for both beginners and advanced users. Highly recommended for anyone looking to streamline their workflow. Check it out here: https://github.com/jjsantos01/jupyter-notebook-mcp.