Confidential guide on numerology and astrology, based of GG33 Public information
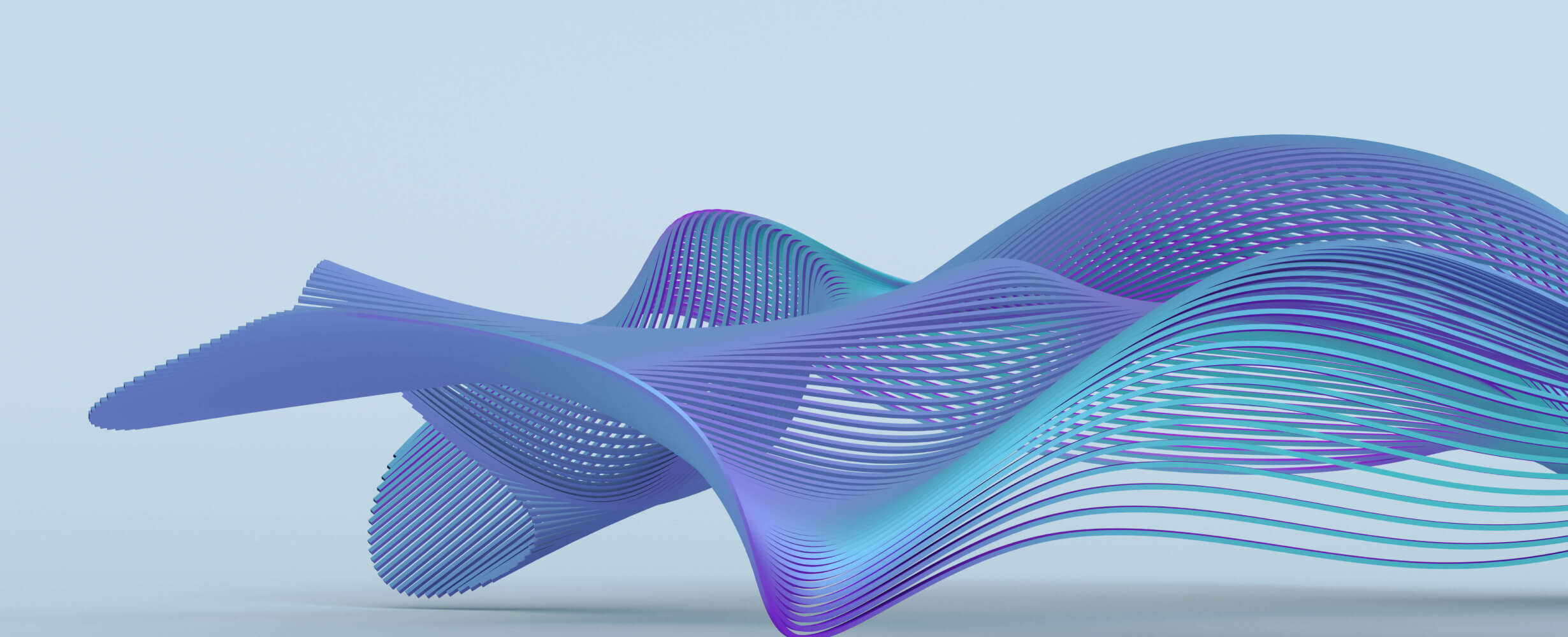
MCP-Chatbot
一个简单的CLI聊天机器人,演示了模型上下文协议(MCP)的集成。
3 years
Works with Finder
2
Github Watches
15
Github Forks
128
Github Stars
MCP Chatbot
This chatbot example demonstrates how to integrate the Model Context Protocol (MCP) into a simple CLI chatbot. The implementation showcases MCP's flexibility by supporting multiple tools through MCP servers and is compatible with any LLM provider that follows OpenAI API standards.
If you find this project helpful, don’t forget to ⭐ star the repository or buy me a ☕ coffee.
Key Features
- LLM Provider Flexibility: Works with any LLM that follows OpenAI API standards (tested with Llama 3.2 90b on Groq and GPT-4o mini on GitHub Marketplace).
- Dynamic Tool Integration: Tools are declared in the system prompt, ensuring maximum compatibility across different LLMs.
- Server Configuration: Supports multiple MCP servers through a simple JSON configuration file like the Claude Desktop App.
Requirements
- Python 3.10
-
python-dotenv
-
requests
-
mcp
-
uvicorn
Installation
-
Clone the repository:
git clone https://github.com/3choff/mcp-chatbot.git cd mcp-chatbot
-
Install the dependencies:
pip install -r requirements.txt
-
Set up environment variables:
Create a
.env
file in the root directory and add your API key:LLM_API_KEY=your_api_key_here
-
Configure servers:
The
servers_config.json
follows the same structure as Claude Desktop, allowing for easy integration of multiple servers. Here's an example:{ "mcpServers": { "sqlite": { "command": "uvx", "args": ["mcp-server-sqlite", "--db-path", "./test.db"] }, "puppeteer": { "command": "npx", "args": ["-y", "@modelcontextprotocol/server-puppeteer"] } } }
Environment variables are supported as well. Pass them as you would with the Claude Desktop App.
Example:
{ "mcpServers": { "server_name": { "command": "uvx", "args": ["mcp-server-name", "--additional-args"], "env": { "API_KEY": "your_api_key_here" } } } }
Usage
-
Run the client:
python main.py
-
Interact with the assistant:
The assistant will automatically detect available tools and can respond to queries based on the tools provided by the configured servers.
-
Exit the session:
Type
quit
orexit
to end the session.
Architecture
- Tool Discovery: Tools are automatically discovered from configured servers.
- System Prompt: Tools are dynamically included in the system prompt, allowing the LLM to understand available capabilities.
- Server Integration: Supports any MCP-compatible server, tested with various server implementations including Uvicorn and Node.js.
Class Structure
- Configuration: Manages environment variables and server configurations
- Server: Handles MCP server initialization, tool discovery, and execution
- Tool: Represents individual tools with their properties and formatting
- LLMClient: Manages communication with the LLM provider
- ChatSession: Orchestrates the interaction between user, LLM, and tools
Logic Flow
flowchart TD
A[Start] --> B[Load Configuration]
B --> C[Initialize Servers]
C --> D[Discover Tools]
D --> E[Format Tools for LLM]
E --> F[Wait for User Input]
F --> G{User Input}
G --> H[Send Input to LLM]
H --> I{LLM Decision}
I -->|Tool Call| J[Execute Tool]
I -->|Direct Response| K[Return Response to User]
J --> L[Return Tool Result]
L --> M[Send Result to LLM]
M --> N[LLM Interprets Result]
N --> O[Present Final Response to User]
K --> O
O --> F
-
Initialization:
- Configuration loads environment variables and server settings
- Servers are initialized with their respective tools
- Tools are discovered and formatted for LLM understanding
-
Runtime Flow:
- User input is received
- Input is sent to LLM with context of available tools
- LLM response is parsed:
- If it's a tool call → execute tool and return result
- If it's a direct response → return to user
- Tool results are sent back to LLM for interpretation
- Final response is presented to user
-
Tool Integration:
- Tools are dynamically discovered from MCP servers
- Tool descriptions are automatically included in system prompt
- Tool execution is handled through standardized MCP protocol
Contributing
Feedback and contributions are welcome. If you encounter any issues or have suggestions for improvements, please create a new issue on the GitHub repository.
If you'd like to contribute to the development of the project, feel free to submit a pull request with your changes.
License
This project is licensed under the MIT License.
相关推荐
Embark on a thrilling diplomatic quest across a galaxy on the brink of war. Navigate complex politics and alien cultures to forge peace and avert catastrophe in this immersive interstellar adventure.
Delivers concise Python code and interprets non-English comments
Reviews
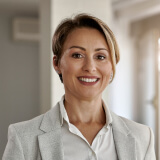
user_Ffy63FsN
The mcp-chatbot by 3choff is truly impressive! Accessible on GitHub (https://github.com/3choff/mcp-chatbot), it excels in natural language understanding and generates accurate, context-aware responses. The seamless integration and smooth user experience make it a top choice for anyone looking to implement an advanced chatbot. Highly recommended!