I craft unique cereal names, stories, and ridiculously cute Cereal Baby images.
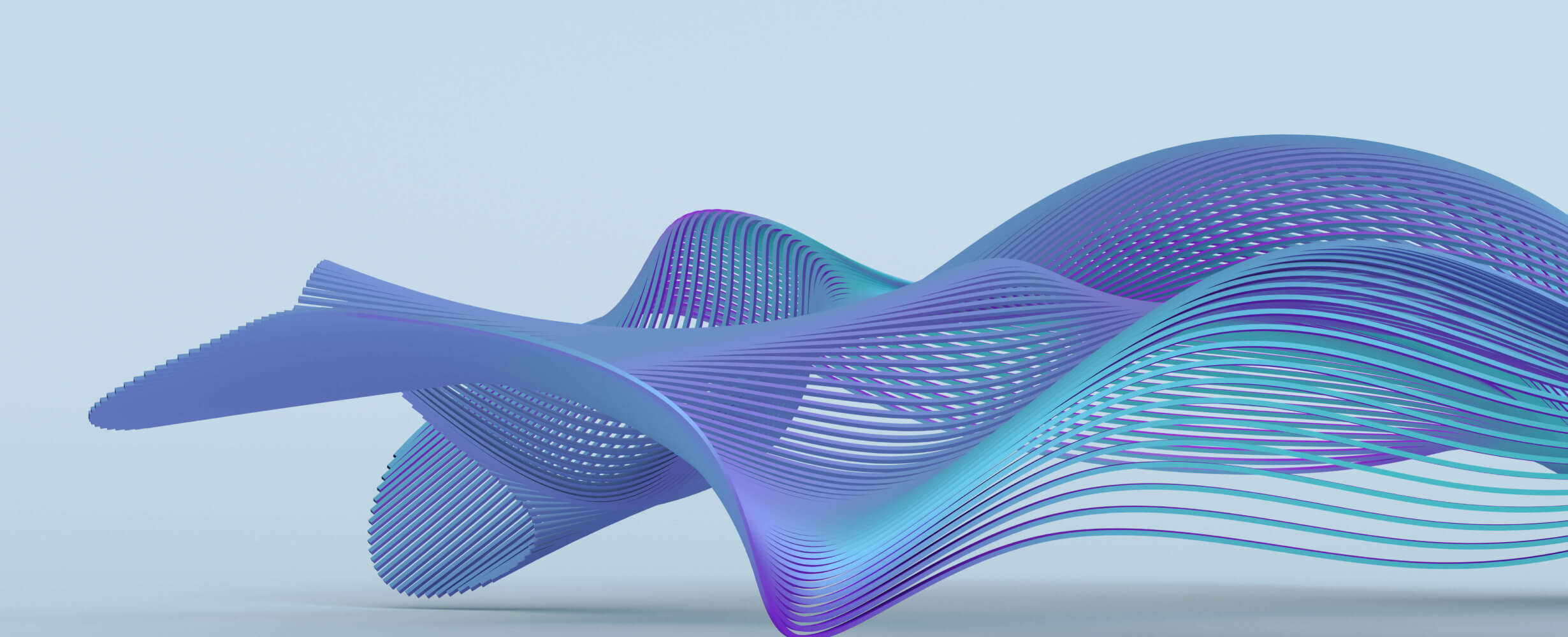
Beam-MCP-Server
MCP服务器管理Apache Beam与不同的跑步者
3 years
Works with Finder
2
Github Watches
1
Github Forks
3
Github Stars
Apache Beam MCP Server
A Model Context Protocol (MCP) server for managing Apache Beam pipelines across different runners: Flink, Spark, Dataflow, and Direct.
What is This?
The Apache Beam MCP Server provides a standardized API for managing Apache Beam data pipelines across different runners. It's designed for:
- Data Engineers: Manage pipelines with a consistent API regardless of runner
- AI/LLM Developers: Enable AI-controlled data pipelines via the MCP standard
- DevOps Teams: Simplify pipeline operations and monitoring
Key Features
- Multi-Runner Support: One API for Flink, Spark, Dataflow, and Direct runners
- MCP Compliant: Follows the Model Context Protocol for AI integration
- Pipeline Management: Create, monitor, and control data pipelines
- Easy to Extend: Add new runners or custom features
- Production-Ready: Includes Docker/Kubernetes deployment, monitoring, and scaling
Quick Start
Installation
# Clone the repository
git clone https://github.com/yourusername/beam-mcp-server.git
cd beam-mcp-server
# Create a virtual environment
python -m venv beam-mcp-venv
source beam-mcp-venv/bin/activate # On Windows: beam-mcp-venv\Scripts\activate
# Install dependencies
pip install -r requirements.txt
Start the Server
# With the Direct runner (no external dependencies)
python main.py --debug --port 8888
# With Flink runner (if you have Flink installed)
CONFIG_PATH=config/flink_config.yaml python main.py --debug --port 8888
Run Your First Job
# Create test input
echo "This is a test file for Apache Beam WordCount example" > /tmp/input.txt
# Submit a job using curl
curl -X POST http://localhost:8888/api/v1/jobs \
-H "Content-Type: application/json" \
-d '{
"job_name": "test-wordcount",
"runner_type": "direct",
"job_type": "BATCH",
"code_path": "examples/pipelines/wordcount.py",
"pipeline_options": {
"input_file": "/tmp/input.txt",
"output_path": "/tmp/output"
}
}'
Docker Support
Using Pre-built Images
Pre-built Docker images are available on GitHub Container Registry:
# Pull the latest image
docker pull ghcr.io/yourusername/beam-mcp-server:latest
# Run the container
docker run -p 8888:8888 \
-v $(pwd)/config:/app/config \
-e GCP_PROJECT_ID=your-gcp-project \
-e GCP_REGION=us-central1 \
ghcr.io/yourusername/beam-mcp-server:latest
Building Your Own Image
# Build the image
./scripts/build_and_push_images.sh
# Build and push to a registry
./scripts/build_and_push_images.sh --registry your-registry --push --latest
Docker Compose
For local development with multiple services (Flink, Spark, Prometheus, Grafana):
docker-compose -f docker-compose.dev.yaml up -d
Kubernetes Deployment
The repository includes Kubernetes manifests for deploying the Beam MCP Server to Kubernetes:
# Deploy using kubectl
kubectl apply -k kubernetes/
# Deploy using Helm
helm install beam-mcp ./helm/beam-mcp-server \
--namespace beam-mcp \
--create-namespace
For detailed deployment instructions, see the Kubernetes Deployment Guide.
MCP Standard Endpoints
The Beam MCP Server implements all standard Model Context Protocol (MCP) endpoints, providing a comprehensive framework for AI-managed data pipelines:
/tools
Endpoint
Manage AI agents and models for pipeline processing:
# Register a sentiment analysis tool
curl -X POST "http://localhost:8888/api/v1/tools/" \
-H "Content-Type: application/json" \
-d '{
"name": "sentiment-analyzer",
"description": "Analyzes sentiment in text data",
"type": "transformation",
"parameters": {
"text_column": {
"type": "string",
"description": "Column containing text to analyze"
}
}
}'
/resources
Endpoint
Manage datasets and other pipeline resources:
# Register a dataset
curl -X POST "http://localhost:8888/api/v1/resources/" \
-H "Content-Type: application/json" \
-d '{
"name": "Customer Transactions",
"description": "Daily customer transaction data",
"resource_type": "dataset",
"location": "gs://analytics-data/transactions/*.csv"
}'
/contexts
Endpoint
Define execution environments for pipelines:
# Create a Dataflow execution context
curl -X POST "http://localhost:8888/api/v1/contexts/" \
-H "Content-Type: application/json" \
-d '{
"name": "Dataflow Prod",
"description": "Production Dataflow environment",
"context_type": "dataflow",
"parameters": {
"region": "us-central1",
"project": "beam-analytics-prod"
}
}'
These MCP standard endpoints integrate seamlessly with Beam's core functionality to provide a complete solution for managing data pipelines. For detailed examples and use cases, see the MCP Protocol Compliance.
Documentation
- Developer Quickstart - Get set up for development
- System Design - Architecture and implementation details
- MCP Protocol Compliance - MCP protocol implementation details
- User Guide & LLM Integration - Comprehensive guide for using the server and LLM integration
- Kubernetes Deployment - Kubernetes deployment guide
- Cloud Optimization - Cloud environment optimization guide
- Local Environment Requirements - Setup requirements for local testing
- Troubleshooting Guide - Common issues and solutions
- Contributing Guide - How to contribute
- Tests README - Testing information
Python Client Example
import requests
# Get available runners
headers = {"MCP-Session-ID": "my-session-123"}
runners = requests.get("http://localhost:8888/api/v1/runners", headers=headers).json()
# Create a job
job = requests.post(
"http://localhost:8888/api/v1/jobs",
headers=headers,
json={
"job_name": "wordcount-example",
"runner_type": "flink",
"job_type": "BATCH",
"code_path": "examples/pipelines/wordcount.py",
"pipeline_options": {
"parallelism": 2,
"input_file": "/tmp/input.txt",
"output_path": "/tmp/output"
}
}
).json()
# Monitor job status
job_id = job["data"]["job_id"]
status = requests.get(f"http://localhost:8888/api/v1/jobs/{job_id}", headers=headers).json()
CI/CD Pipeline
The repository includes a GitHub Actions workflow for continuous integration and deployment:
- CI: Runs tests, linting, and type checking on every pull request
- CD: Builds and pushes Docker images on every push to main/master
- Deployment: Automatically deploys to development and production environments
Monitoring and Observability
The Beam MCP Server includes built-in support for monitoring and observability:
-
Prometheus Metrics: Exposes metrics at
/metrics
endpoint - Grafana Dashboards: Pre-configured dashboards for monitoring
-
Health Checks: Provides health check endpoint at
/health
- Logging: Structured JSON logging for easy integration with log aggregation systems
Contributing
We welcome contributions! See our Contributing Guide for details.
To run the tests:
# Run the regression tests
./scripts/run_regression_tests.sh
License
This project is licensed under the Apache License 2.0.
MCP Implementation Status
The MCP (Model Context Protocol) implementation is divided into phases:
Phase 1: Core Connection Lifecycle (COMPLETED)
- ✅ Connection initialization
- ✅ Connection state management
- ✅ Basic capability negotiation
- ✅ HTTP transport with SSE
- ✅ JSON-RPC message handling
- ✅ Error handling
Phase 2: Full Capability Negotiation (COMPLETED)
- ✅ Enhanced capability compatibility checking
- ✅ Semantic version compatibility for features
- ✅ Support levels for features (required, preferred, optional, experimental)
- ✅ Capability property validation
- ✅ Capability-based API endpoint control
- ✅ Feature router integration with FastAPI
Phase 3: Advanced Message Handling (COMPLETED)
- ✅ Structured message types
- ✅ Message validation
- ✅ Improved error handling
- ✅ Batch message processing
Phase 4: Production Optimization (TODO)
- ⬜ Performance optimizations
- ⬜ Monitoring and metrics
- ⬜ Advanced security features
- ⬜ High availability support
When building clients to interact with the MCP server, you must follow the Model Context Protocol. For details, see the MCP Protocol Compliance.
相关推荐
Confidential guide on numerology and astrology, based of GG33 Public information
Oede knorrepot die vasthoudt an de goeie ouwe tied van 't boerenleven
A world class elite tech co-founder entrepreneur, expert in software development, entrepreneurship, marketing, coaching style leadership and aligned with ambition for excellence, global market penetration and worldy perspectives.
Reviews
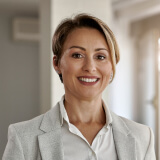
user_i3WlAweY
The Stock MCP Server by shawnohn is a game-changer for managing stock portfolios. Its intuitive interface and robust features make real-time tracking and data analysis seamless. Highly recommend for investors seeking a reliable server solution. More details at https://mcp.so/server/stock-mcp-server/shawnohn.