I craft unique cereal names, stories, and ridiculously cute Cereal Baby images.
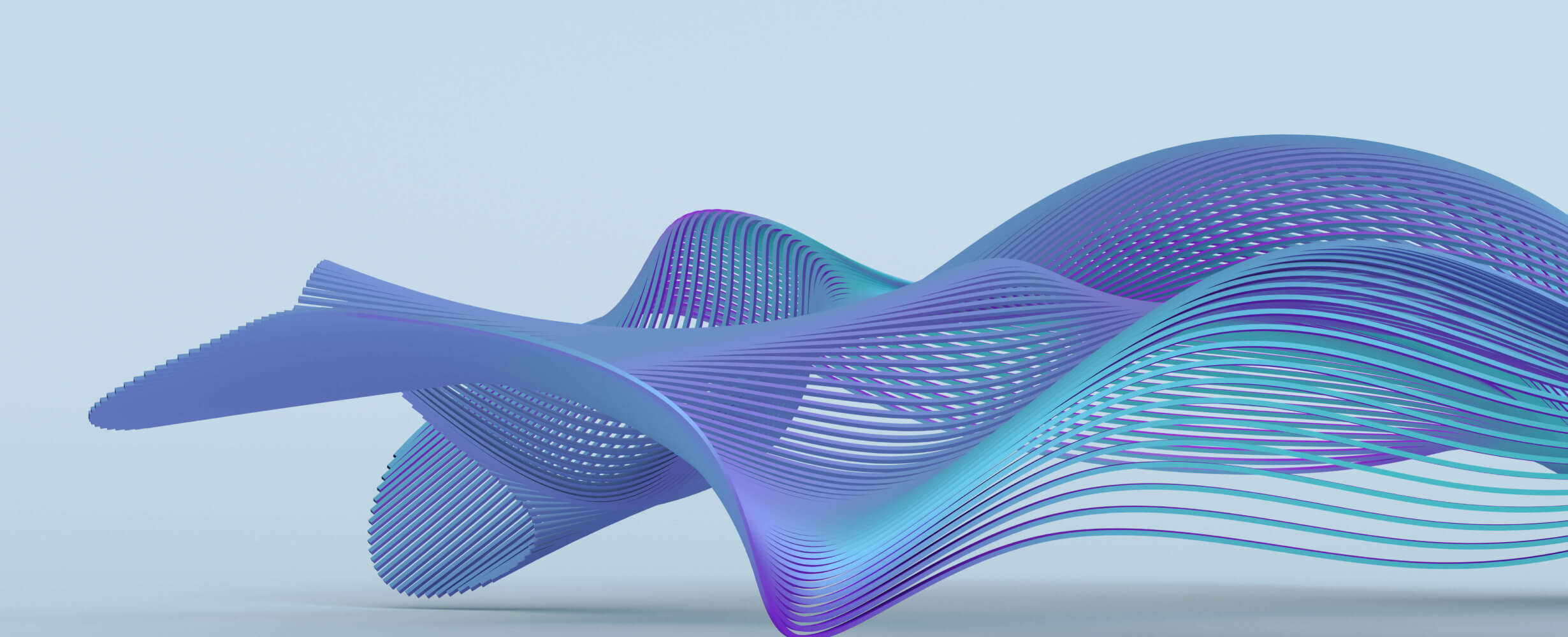
不整洁的MCP服务器
一个用于不舒服的MCP服务器 - 一个使LLM微调2倍的库,存储器的记忆少80%
3 years
Works with Finder
1
Github Watches
2
Github Forks
2
Github Stars
Unsloth MCP Server
An MCP server for Unsloth - a library that makes LLM fine-tuning 2x faster with 80% less memory.
What is Unsloth?
Unsloth is a library that dramatically improves the efficiency of fine-tuning large language models:
- Speed: 2x faster fine-tuning compared to standard methods
- Memory: 80% less VRAM usage, allowing fine-tuning of larger models on consumer GPUs
- Context Length: Up to 13x longer context lengths (e.g., 89K tokens for Llama 3.3 on 80GB GPUs)
- Accuracy: No loss in model quality or performance
Unsloth achieves these improvements through custom CUDA kernels written in OpenAI's Triton language, optimized backpropagation, and dynamic 4-bit quantization.
Features
- Optimize fine-tuning for Llama, Mistral, Phi, Gemma, and other models
- 4-bit quantization for efficient training
- Extended context length support
- Simple API for model loading, fine-tuning, and inference
- Export to various formats (GGUF, Hugging Face, etc.)
Quick Start
- Install Unsloth:
pip install unsloth
- Install and build the server:
cd unsloth-server npm install npm run build
- Add to MCP settings:
{ "mcpServers": { "unsloth-server": { "command": "node", "args": ["/path/to/unsloth-server/build/index.js"], "env": { "HUGGINGFACE_TOKEN": "your_token_here" // Optional }, "disabled": false, "autoApprove": [] } } }
Available Tools
check_installation
Verify if Unsloth is properly installed on your system.
Parameters: None
Example:
const result = await use_mcp_tool({
server_name: "unsloth-server",
tool_name: "check_installation",
arguments: {}
});
list_supported_models
Get a list of all models supported by Unsloth, including Llama, Mistral, Phi, and Gemma variants.
Parameters: None
Example:
const result = await use_mcp_tool({
server_name: "unsloth-server",
tool_name: "list_supported_models",
arguments: {}
});
load_model
Load a pretrained model with Unsloth optimizations for faster inference and fine-tuning.
Parameters:
-
model_name
(required): Name of the model to load (e.g., "unsloth/Llama-3.2-1B") -
max_seq_length
(optional): Maximum sequence length for the model (default: 2048) -
load_in_4bit
(optional): Whether to load the model in 4-bit quantization (default: true) -
use_gradient_checkpointing
(optional): Whether to use gradient checkpointing to save memory (default: true)
Example:
const result = await use_mcp_tool({
server_name: "unsloth-server",
tool_name: "load_model",
arguments: {
model_name: "unsloth/Llama-3.2-1B",
max_seq_length: 4096,
load_in_4bit: true
}
});
finetune_model
Fine-tune a model with Unsloth optimizations using LoRA/QLoRA techniques.
Parameters:
-
model_name
(required): Name of the model to fine-tune -
dataset_name
(required): Name of the dataset to use for fine-tuning -
output_dir
(required): Directory to save the fine-tuned model -
max_seq_length
(optional): Maximum sequence length for training (default: 2048) -
lora_rank
(optional): Rank for LoRA fine-tuning (default: 16) -
lora_alpha
(optional): Alpha for LoRA fine-tuning (default: 16) -
batch_size
(optional): Batch size for training (default: 2) -
gradient_accumulation_steps
(optional): Number of gradient accumulation steps (default: 4) -
learning_rate
(optional): Learning rate for training (default: 2e-4) -
max_steps
(optional): Maximum number of training steps (default: 100) -
dataset_text_field
(optional): Field in the dataset containing the text (default: 'text') -
load_in_4bit
(optional): Whether to use 4-bit quantization (default: true)
Example:
const result = await use_mcp_tool({
server_name: "unsloth-server",
tool_name: "finetune_model",
arguments: {
model_name: "unsloth/Llama-3.2-1B",
dataset_name: "tatsu-lab/alpaca",
output_dir: "./fine-tuned-model",
max_steps: 100,
batch_size: 2,
learning_rate: 2e-4
}
});
generate_text
Generate text using a fine-tuned Unsloth model.
Parameters:
-
model_path
(required): Path to the fine-tuned model -
prompt
(required): Prompt for text generation -
max_new_tokens
(optional): Maximum number of tokens to generate (default: 256) -
temperature
(optional): Temperature for text generation (default: 0.7) -
top_p
(optional): Top-p for text generation (default: 0.9)
Example:
const result = await use_mcp_tool({
server_name: "unsloth-server",
tool_name: "generate_text",
arguments: {
model_path: "./fine-tuned-model",
prompt: "Write a short story about a robot learning to paint:",
max_new_tokens: 512,
temperature: 0.8
}
});
export_model
Export a fine-tuned Unsloth model to various formats for deployment.
Parameters:
-
model_path
(required): Path to the fine-tuned model -
export_format
(required): Format to export to (gguf, ollama, vllm, huggingface) -
output_path
(required): Path to save the exported model -
quantization_bits
(optional): Bits for quantization (for GGUF export) (default: 4)
Example:
const result = await use_mcp_tool({
server_name: "unsloth-server",
tool_name: "export_model",
arguments: {
model_path: "./fine-tuned-model",
export_format: "gguf",
output_path: "./exported-model.gguf",
quantization_bits: 4
}
});
Advanced Usage
Custom Datasets
You can use custom datasets by formatting them properly and hosting them on Hugging Face or providing a local path:
const result = await use_mcp_tool({
server_name: "unsloth-server",
tool_name: "finetune_model",
arguments: {
model_name: "unsloth/Llama-3.2-1B",
dataset_name: "json",
data_files: {"train": "path/to/your/data.json"},
output_dir: "./fine-tuned-model"
}
});
Memory Optimization
For large models on limited hardware:
- Reduce batch size and increase gradient accumulation steps
- Use 4-bit quantization
- Enable gradient checkpointing
- Reduce sequence length if possible
Troubleshooting
Common Issues
- CUDA Out of Memory: Reduce batch size, use 4-bit quantization, or try a smaller model
- Import Errors: Ensure you have the correct versions of torch, transformers, and unsloth installed
- Model Not Found: Check that you're using a supported model name or have access to private models
Version Compatibility
- Python: 3.10, 3.11, or 3.12 (not 3.13)
- CUDA: 11.8 or 12.1+ recommended
- PyTorch: 2.0+ recommended
Performance Benchmarks
Model | VRAM | Unsloth Speed | VRAM Reduction | Context Length |
---|---|---|---|---|
Llama 3.3 (70B) | 80GB | 2x faster | >75% | 13x longer |
Llama 3.1 (8B) | 80GB | 2x faster | >70% | 12x longer |
Mistral v0.3 (7B) | 80GB | 2.2x faster | 75% less | - |
Requirements
- Python 3.10-3.12
- NVIDIA GPU with CUDA support (recommended)
- Node.js and npm
License
Apache-2.0
相关推荐
Evaluator for marketplace product descriptions, checks for relevancy and keyword stuffing.
Confidential guide on numerology and astrology, based of GG33 Public information
A geek-themed horoscope generator blending Bitcoin prices, tech jargon, and astrological whimsy.
Therapist adept at identifying core issues and offering practical advice with images.
Reviews
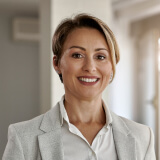
user_2sR6dT5a
The MCP Server Docker Image for Choreo, authored by pcnfernando, is a robust solution for developers. It streamlines the deployment process, ensuring smooth and efficient container orchestration. Highly recommend for anyone seeking to optimize their server hosting experience. Check it out at https://mcp.so/server/mcp-server-hosting/pcnfernando.