I craft unique cereal names, stories, and ridiculously cute Cereal Baby images.
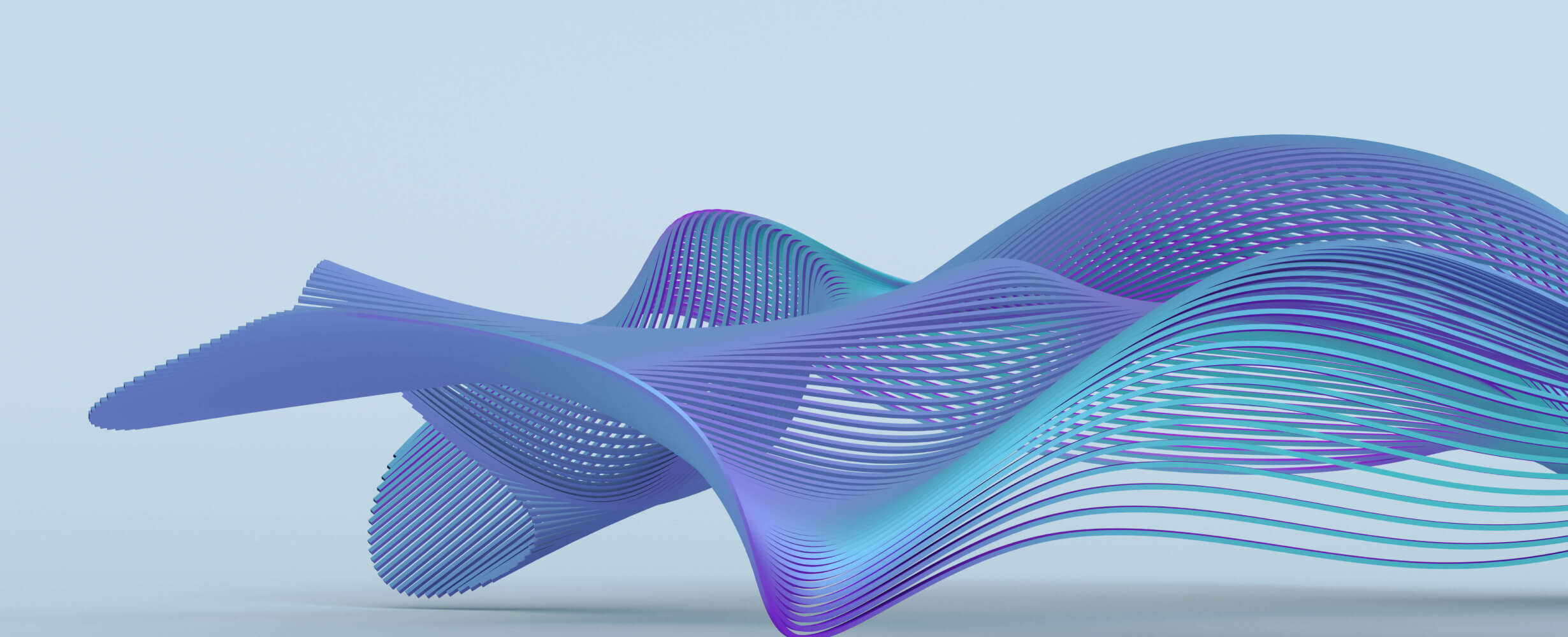
mcp-openvision
MCP Server using OpenRouter models to get descriptions for images
3 years
Works with Finder
1
Github Watches
0
Github Forks
3
Github Stars
MCP OpenVision
Overview
MCP OpenVision is a Model Context Protocol (MCP) server that provides image analysis capabilities powered by OpenRouter vision models. It enables AI assistants to analyze images via a simple interface within the MCP ecosystem.
Installation
Installing via Smithery
To install mcp-openvision for Claude Desktop automatically via Smithery:
npx -y @smithery/cli install @Nazruden/mcp-openvision --client claude
Using pip
pip install mcp-openvision
Using UV (recommended)
uv pip install mcp-openvision
Configuration
MCP OpenVision requires an OpenRouter API key and can be configured through environment variables:
- OPENROUTER_API_KEY (required): Your OpenRouter API key
- OPENROUTER_DEFAULT_MODEL (optional): The vision model to use
OpenRouter Vision Models
MCP OpenVision works with any OpenRouter model that supports vision capabilities. The default model is qwen/qwen2.5-vl-32b-instruct:free
, but you can specify any other compatible model.
Some popular vision models available through OpenRouter include:
-
qwen/qwen2.5-vl-32b-instruct:free
(default) -
anthropic/claude-3-5-sonnet
-
anthropic/claude-3-opus
-
anthropic/claude-3-sonnet
-
openai/gpt-4o
You can specify custom models by setting the OPENROUTER_DEFAULT_MODEL
environment variable or by passing the model
parameter directly to the image_analysis
function.
Usage
Testing with MCP Inspector
The easiest way to test MCP OpenVision is with the MCP Inspector tool:
npx @modelcontextprotocol/inspector uvx mcp-openvision
Integration with Claude Desktop or Cursor
-
Edit your MCP configuration file:
- Windows:
%USERPROFILE%\.cursor\mcp.json
- macOS:
~/.cursor/mcp.json
or~/Library/Application Support/Claude/claude_desktop_config.json
- Windows:
-
Add the following configuration:
{
"mcpServers": {
"openvision": {
"command": "uvx",
"args": ["mcp-openvision"],
"env": {
"OPENROUTER_API_KEY": "your_openrouter_api_key_here",
"OPENROUTER_DEFAULT_MODEL": "anthropic/claude-3-sonnet"
}
}
}
}
Running Locally for Development
# Set the required API key
export OPENROUTER_API_KEY="your_api_key"
# Run the server module directly
python -m mcp_openvision
Features
MCP OpenVision provides the following core tool:
-
image_analysis: Analyze images with vision models, supporting various parameters:
-
image
: Can be provided as:- Base64-encoded image data
- Image URL (http/https)
- Local file path
-
query
: User instruction for the image analysis task -
system_prompt
: Instructions that define the model's role and behavior (optional) -
model
: Vision model to use -
temperature
: Controls randomness (0.0-1.0) -
max_tokens
: Maximum response length
-
Crafting Effective Queries
The query
parameter is crucial for getting useful results from the image analysis. A well-crafted query provides context about:
- Purpose: Why you're analyzing this image
- Focus areas: Specific elements or details to pay attention to
- Required information: The type of information you need to extract
- Format preferences: How you want the results structured
Examples of Effective Queries
Basic Query | Enhanced Query |
---|---|
"Describe this image" | "Identify all retail products visible in this store shelf image and estimate their price range" |
"What's in this image?" | "Analyze this medical scan for abnormalities, focusing on the highlighted area and providing possible diagnoses" |
"Analyze this chart" | "Extract the numerical data from this bar chart showing quarterly sales, and identify the key trends from 2022-2023" |
"Read the text" | "Transcribe all visible text in this restaurant menu, preserving the item names, descriptions, and prices" |
By providing context about why you need the analysis and what specific information you're seeking, you help the model focus on relevant details and produce more valuable insights.
Example Usage
# Analyze an image from a URL
result = await image_analysis(
image="https://example.com/image.jpg",
query="Describe this image in detail"
)
# Analyze an image from a local file with a focused query
result = await image_analysis(
image="path/to/local/image.jpg",
query="Identify all traffic signs in this street scene and explain their meanings for a driver education course"
)
# Analyze with a base64-encoded image and a specific analytical purpose
result = await image_analysis(
image="SGVsbG8gV29ybGQ=...", # base64 data
query="Examine this product packaging design and highlight elements that could be improved for better visibility and brand recognition"
)
# Customize the system prompt for specialized analysis
result = await image_analysis(
image="path/to/local/image.jpg",
query="Analyze the composition and artistic techniques used in this painting, focusing on how they create emotional impact",
system_prompt="You are an expert art historian with deep knowledge of painting techniques and art movements. Focus on formal analysis of composition, color, brushwork, and stylistic elements."
)
Image Input Types
The image_analysis
tool accepts several types of image inputs:
- Base64-encoded strings
- Image URLs - must start with http:// or https://
-
File paths:
- Absolute paths: full paths starting with / (Unix) or drive letter (Windows)
- Relative paths: paths relative to the current working directory
-
Relative paths with project_root: use the
project_root
parameter to specify a base directory
Using Relative Paths
When using relative file paths (like "examples/image.jpg"), you have two options:
- The path must be relative to the current working directory where the server is running
- Or, you can specify a
project_root
parameter:
# Example with relative path and project_root
result = await image_analysis(
image="examples/image.jpg",
project_root="/path/to/your/project",
query="What is in this image?"
)
This is particularly useful in applications where the current working directory may not be predictable or when you want to reference files using paths relative to a specific directory.
Development
Setup Development Environment
# Clone the repository
git clone https://github.com/modelcontextprotocol/mcp-openvision.git
cd mcp-openvision
# Install development dependencies
pip install -e ".[dev]"
Code Formatting
This project uses Black for automatic code formatting. The formatting is enforced through GitHub Actions:
- All code pushed to the repository is automatically formatted with Black
- For pull requests from repository collaborators, Black formats the code and commits directly to the PR branch
- For pull requests from forks, Black creates a new PR with the formatted code that can be merged into the original PR
You can also run Black locally to format your code before committing:
# Format all Python code in the src and tests directories
black src tests
Run Tests
pytest
Release Process
This project uses an automated release process:
- Update the version in
pyproject.toml
following Semantic Versioning principles- You can use the helper script:
python scripts/bump_version.py [major|minor|patch]
- You can use the helper script:
- Update the
CHANGELOG.md
with details about the new version- The script also creates a template entry in CHANGELOG.md that you can fill in
- Commit and push these changes to the
main
branch - The GitHub Actions workflow will:
- Detect the version change
- Automatically create a new GitHub release
- Trigger the publishing workflow that publishes to PyPI
This automation helps maintain a consistent release process and ensures that every release is properly versioned and documented.
Support
If you find this project helpful, consider buying me a coffee to support ongoing development and maintenance.
License
This project is licensed under the MIT License - see the LICENSE file for details.
相关推荐
Evaluator for marketplace product descriptions, checks for relevancy and keyword stuffing.
Confidential guide on numerology and astrology, based of GG33 Public information
Converts Figma frames into front-end code for various mobile frameworks.
Advanced software engineer GPT that excels through nailing the basics.
Take an adjectivised noun, and create images making it progressively more adjective!
Discover the most comprehensive and up-to-date collection of MCP servers in the market. This repository serves as a centralized hub, offering an extensive catalog of open-source and proprietary MCP servers, complete with features, documentation links, and contributors.
Micropython I2C-based manipulation of the MCP series GPIO expander, derived from Adafruit_MCP230xx
Mirror ofhttps://github.com/agentience/practices_mcp_server
Mirror ofhttps://github.com/bitrefill/bitrefill-mcp-server
An AI chat bot for small and medium-sized teams, supporting models such as Deepseek, Open AI, Claude, and Gemini. 专为中小团队设计的 AI 聊天应用,支持 Deepseek、Open AI、Claude、Gemini 等模型。
Bridge between Ollama and MCP servers, enabling local LLMs to use Model Context Protocol tools
Reviews
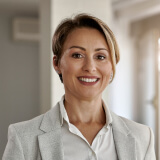
user_q33JP0Ej
As a dedicated user of mcp application, I wholeheartedly recommend mcp-openvision by Nazruden. This open-source tool available on GitHub provides exceptional functionality and supports multiple languages, enhancing productivity and usability. Whether you're starting with the provided URL or exploring its comprehensive features, mcp-openvision consistently delivers a seamless experience. Highly worth checking out!