I craft unique cereal names, stories, and ridiculously cute Cereal Baby images.
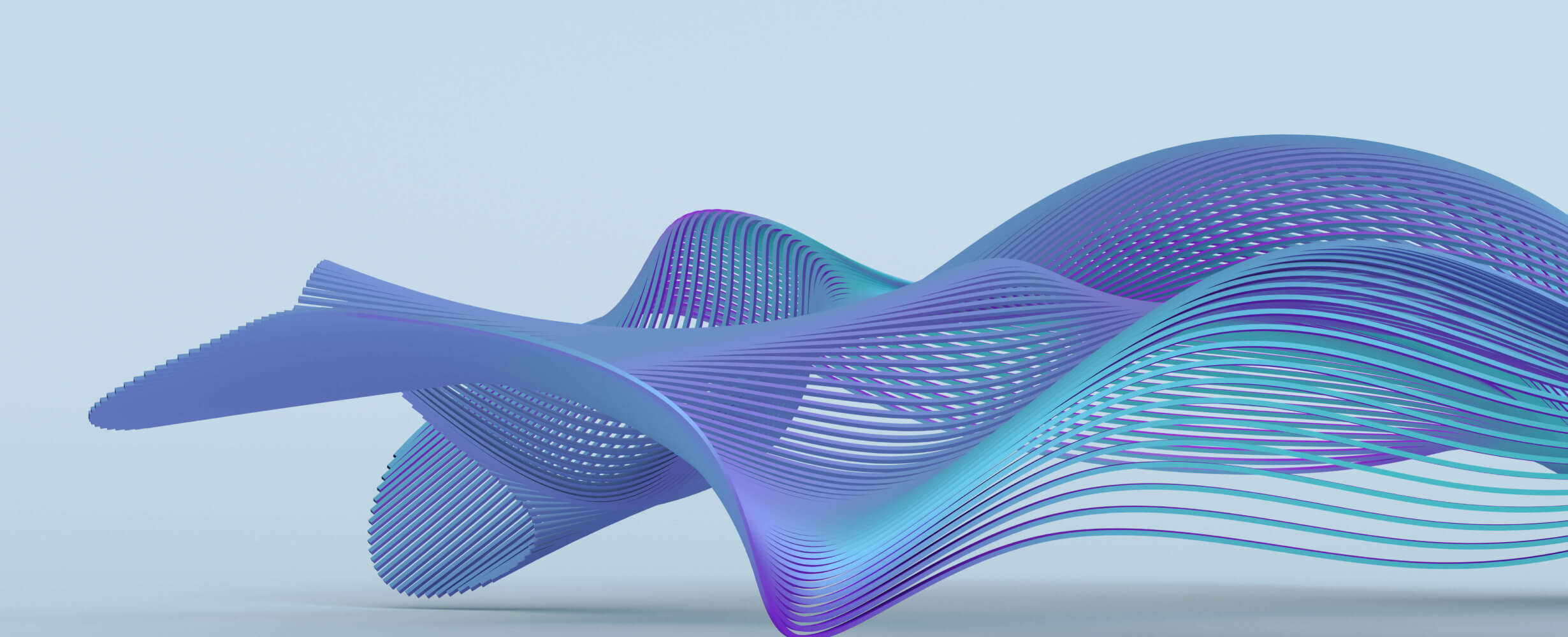
langgraph-mcp-integration
MCP architecture demo with single-server & multi-server clients using LangGraph, AI-driven tool calls, and async communication.
3 years
Works with Finder
1
Github Watches
0
Github Forks
0
Github Stars
Understanding MCP Architecture: Single-Server vs Multi-Server Clients
Modern AI-driven applications require efficient and scalable architectures to interact with multiple external services. The Multi-Client Protocol (MCP) provides a robust framework to communicate with various tool servers, enabling seamless interaction between AI models and external computations. In this blog, we'll explore the advantages of MCP architecture by comparing single-server and multi-server clients.
Math Server (math_server.py
)
The Math Server acts as an MCP tool server that provides basic mathematical operations like addition and multiplication. It listens for incoming requests, processes tool invocations, and responds with results.
Code Breakdown
from mcp.server.fastmcp import FastMCP
mcp = FastMCP("Math")
@mcp.tool()
def add(a: int, b: int) -> int:
"""Add two numbers"""
return a + b
@mcp.tool()
def multiply(a: int, b: int) -> int:
"""Multiply two numbers"""
return a * b
if __name__ == "__main__":
mcp.run(transport="stdio")
- Defines an MCP server named Math.
- Registers
add(a, b)
andmultiply(a, b)
as available tools. - Runs with stdio transport, allowing communication via standard input/output.
Single-Server Client
A single-server client connects to one MCP server, which exposes a set of tools to be used in computations. Let's analyze the single_server_client.py
script, which interacts with math_server.py
to perform mathematical operations.
How It Works
-
Start the Math Server: The
math_server.py
runs as an MCP server exposing two tools:add(a, b)
andmultiply(a, b)
. - Initialize a Stdio Connection: The client creates a subprocess to communicate with the math server via stdio transport.
-
Load Available Tools: The client fetches the available tools (
add
,multiply
) from the server. -
Invoke AI Model: The AI model,
GPT-4o
, uses LangGraph’s ReAct (Reasoning + Acting) agent to decide how to solve the given mathematical expression. -
Perform Computation: The model generates tool calls, invoking
add(3,5)
first, thenmultiply(8,12)
, and finally returns the computed answer.
Code Breakdown (single_server_client.py)
server_params = StdioServerParameters(
command="python",
args=["server/math_server.py"],
)
async with stdio_client(server_params) as (read, write):
async with ClientSession(read, write) as session:
await session.initialize()
tools = await load_mcp_tools(session)
agent = create_react_agent(model, tools)
events = await agent.ainvoke({"messages": "what's (3 + 5) x 12?"})
The output prints:
----------------------------------------------------------------------------
The result is 96
MCP Communication Flow (Sequence Diagram)
sequenceDiagram
participant Client as Client Script
participant LangGraph as LangGraph React Agent
participant ClientSession as MCP ClientSession
participant stdio as stdio Transport Layer
participant MathServer as Math Server Process
participant Tools as Math Tools (add/multiply)
participant LLM as GPT-4o Model
Note over Client: Starts execution with asyncio.run()
Client->>MathServer: Launch as subprocess with stdio parameters
Client->>stdio: Create stdio client connection
stdio-->>Client: Return read/write streams
Client->>ClientSession: Initialize session
ClientSession->>stdio: Send initialize message
stdio->>MathServer: Forward initialize request
MathServer-->>stdio: Return server capabilities
stdio-->>ClientSession: Return initialization response
ClientSession-->>Client: Session initialized
Client->>ClientSession: load_mcp_tools()
ClientSession->>stdio: Request available tools
stdio->>MathServer: Forward tools request
MathServer->>Tools: Discover registered tools (add, multiply)
Tools-->>MathServer: Return tool descriptions
MathServer-->>stdio: Return tool descriptions
stdio-->>ClientSession: Return tool descriptions
ClientSession-->>Client: Return langchain-compatible tools
Client->>LangGraph: Create React agent with model and tools
LangGraph-->>Client: Return agent instance
Client->>LangGraph: agent.ainvoke("what's (3 + 5) x 12?")
LangGraph->>LLM: Generate reasoning and tool calls
Note over LLM: Decides to use add(3, 5) first
LLM-->>LangGraph: Return reasoning and add(3, 5) tool call
LangGraph->>ClientSession: Call add(3, 5)
ClientSession->>stdio: Send tool execution request
stdio->>MathServer: Forward tool execution request
MathServer->>Tools: Execute add(3, 5)
Tools-->>MathServer: Return result (8)
MathServer-->>stdio: Return tool execution result
stdio-->>ClientSession: Return tool execution result
ClientSession-->>LangGraph: Return add result (8)
LangGraph->>LLM: Generate next reasoning step with add result
Note over LLM: Decides to use multiply(8, 12) next
LLM-->>LangGraph: Return reasoning and multiply(8, 12) tool call
LangGraph->>ClientSession: Call multiply(8, 12)
ClientSession->>stdio: Send tool execution request
stdio->>MathServer: Forward tool execution request
MathServer->>Tools: Execute multiply(8, 12)
Tools-->>MathServer: Return result (96)
MathServer-->>stdio: Return tool execution result
stdio-->>ClientSession: Return tool execution result
ClientSession-->>LangGraph: Return multiply result (96)
LangGraph->>LLM: Generate final answer with all results
LLM-->>LangGraph: Return final answer "The result is 96"
LangGraph-->>Client: Return complete event trace and answer
Client->>Client: Print final answer
Weather Server (weather_server.py
)
The Weather Server is another MCP tool server that provides real-time weather information. It listens for incoming weather queries and responds with the latest weather data.
Command to start the server:
python server/weather_server.py
Code Breakdown
from mcp.server.fastmcp import FastMCP
mcp = FastMCP("Weather")
@mcp.tool()
async def get_weather(location: str) -> str:
"""Get weather for location."""
return "It's always sunny in New York"
if __name__ == "__main__":
mcp.run(transport="sse")
- Defines an MCP server named Weather.
- Registers
get_weather(location)
as an available tool. - Runs with SSE (Server-Sent Events) transport, allowing real-time event streaming.
Multi-Server Client
A multi-server client can interact with multiple MCP servers simultaneously. The multi_server_client.py
script demonstrates this by communicating with both math_server.py
and weather_server.py
.
How It Works
-
Initialize Multiple Servers: The client connects to the Math server using
stdio
and the Weather server via SSE (Server-Sent Events). - Load Tools from Multiple Servers: The AI model gains access to tools from both servers.
- Parallel Processing: The client can now invoke tools from different servers within the same execution.
- AI-driven Responses: The model processes both math expressions and weather queries using multiple MCP servers.
Code Breakdown (multi_server_client.py)
async with MultiServerMCPClient(
{
"math": {
"command": "python",
"args": ["server/math_server.py"],
"transport": "stdio",
},
"weather": {
"url": "http://localhost:8000/sse",
"transport": "sse",
},
}
) as client:
agent = create_react_agent(model, client.get_tools())
math_response = await agent.ainvoke({"messages": "what's (3 + 5) x 12?"})
weather_response = await agent.ainvoke({"messages": "what is the weather in nyc?"})
Multi-Server Client Response
The output from the multi-server client looks like this:
{
"math_response": "The result of (3 + 5) x 12 is 96.",
"weather_response": "The weather in NYC is currently sunny."
}
Benefits of MCP Architecture
- Scalability: Easily extendable to multiple services.
- Parallel Execution: Allows concurrent queries to different servers.
-
Flexible Communication: Supports multiple transport layers (
stdio
,sse
, etc.). - AI Integration: Seamlessly connects AI models with external computation services.
Code Setup and Execution
To clone and execute the code, follow these steps:
git clone https://github.com/commitbyrajat/langgraph-mcp-integration.git
cd langgraph-mcp-integration
rye sync # Sync dependencies
rye run python server/weather_server.py &
rye run python client/single_server_client.py
rye run python client/multi_server_client.py
MCP architecture enables developers to build powerful AI-driven applications that interact with multiple services efficiently. 🚀
相关推荐
Evaluator for marketplace product descriptions, checks for relevancy and keyword stuffing.
Confidential guide on numerology and astrology, based of GG33 Public information
Converts Figma frames into front-end code for various mobile frameworks.
Advanced software engineer GPT that excels through nailing the basics.
Take an adjectivised noun, and create images making it progressively more adjective!
Discover the most comprehensive and up-to-date collection of MCP servers in the market. This repository serves as a centralized hub, offering an extensive catalog of open-source and proprietary MCP servers, complete with features, documentation links, and contributors.
Micropython I2C-based manipulation of the MCP series GPIO expander, derived from Adafruit_MCP230xx
Mirror ofhttps://github.com/agentience/practices_mcp_server
Mirror ofhttps://github.com/bitrefill/bitrefill-mcp-server
An AI chat bot for small and medium-sized teams, supporting models such as Deepseek, Open AI, Claude, and Gemini. 专为中小团队设计的 AI 聊天应用,支持 Deepseek、Open AI、Claude、Gemini 等模型。
Bridge between Ollama and MCP servers, enabling local LLMs to use Model Context Protocol tools
Reviews
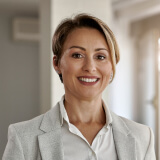
user_56l83rGB
As a devoted user of the MCP application, I highly recommend the langgraph-mcp-integration by commitbyrajat. This seamless integration improves our workflow significantly by providing enhanced graph visualization and analysis capabilities. It’s user-friendly and incredibly efficient. Check it out on GitHub: https://github.com/commitbyrajat/langgraph-mcp-integration.