Create and Publish Business Websites in seconds. AI will gather all the details about your website and generate link to your website.
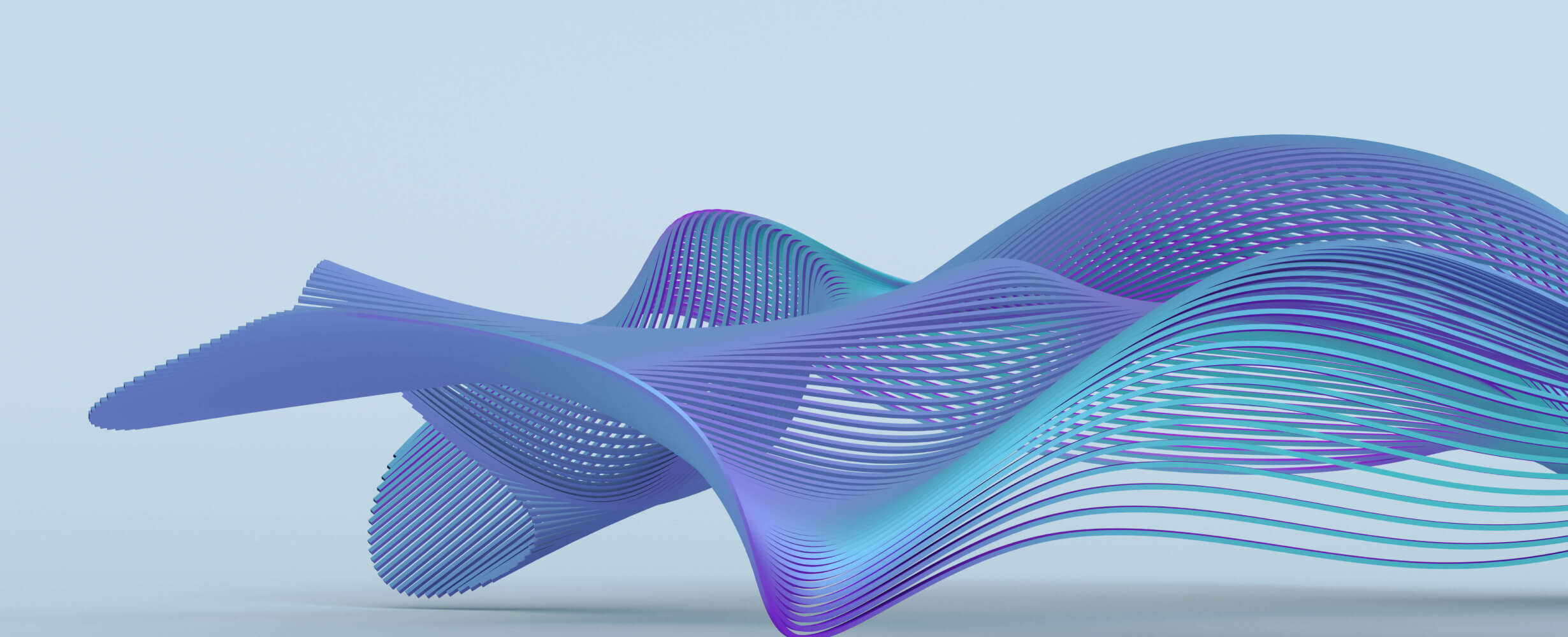
Baml-Agents
Gebäudeagenten mit BAML für die strukturierte Generation mit LLMs, MCP-Tools und 12-Faktor-Agenten-Prinzipien
3
Github Watches
0
Github Forks
3
Github Stars
baml‑agents
Building Agents with BAML for structured generation with LLMs, MCP Tools, and 12-Factor Agents principles
This repository shares useful patterns I use when working with BAML. The API is unstable and may change in future versions. Install with:
pip install "baml-agents>=0.8.0,<0.9.0"
Contents
-
Flexible LLM Client Management in BAML
- Effortlessly switch between different LLM providers (like OpenAI, Anthropic, Google) at runtime using simple helper functions.
- Bridge compatibility gaps: Connect to unsupported LLM backends or tracing systems (e.g., Langfuse, LangSmith) via standard proxy setups.
- Solve common configuration issues: Learn alternatives for managing API keys and client settings if environment variables aren't suitable.
-
Introduction to AI Tool Use with BAML
- Learn how to define custom actions (tools) for your AI using Pydantic models, making your agents capable of doing things.
- See how to integrate these tools with BAML manually or dynamically using
ActionRunner
for flexible structured outputs. - Understand how BAML translates goals into structured LLM calls that select and utilize the appropriate tool.
-
Integrating Standardized MCP Tools with BAML
- Discover how to leverage the Model Context Protocol (MCP) to easily plug-and-play pre-built 3rd party tools (like calculators, web search) into your BAML agents.
- See
ActionRunner
in action, automatically discovering and integrating tools from MCP servers with minimal configuration. - Learn techniques to filter and select specific MCP tools to offer to the LLM, controlling the agent's capabilities precisely.
-
Interactive BAML Development in Jupyter
- See BAML's structured data generation stream live into your Jupyter output cell as the LLM generates it.
- Interactively inspect the details: Use collapsible sections to view full LLM prompts and responses, optionally grouped by call or session, directly in the notebook.
- Chat with your agent: Interactive chat widget right in the notebook, allowing you to chat with your agent in real-time.
-
Simple Agent Demonstration
- Putting it all together: Build a simple, functional agent capable of tackling a multi-step task.
- Learn how to combine custom Python actions (defined as
Action
classes) with standardized MCP tools (like calculators or time servers) managed byActionRunner
. - Follow the agent's decision-making loop driven by BAML's structured output generation (
GetNextAction
), see it execute tools, and observe how it uses the results to progress. - Includes demonstration of
JupyterBamlMonitor
for transparent inspection of the underlying LLM interactions.
Simple example
[!TIP] The code below is trimmed for brevity to illustrate the core concepts. Some function names or setup steps may differ slightly from the full notebook implementation for clarity in this example. The full, runnable code is available in the notebook Simple Agent Demonstration (notebooks/05_simple_agent_demo.ipynb)
Show code for the example below
def get_weather_info(city: str):
return f"The weather in {city} is 63 degrees fahrenheit with cloudy conditions."
def stop_execution(final_answer: str):
return f"Final answer: {final_answer}"
r = ActionRunner() # Doing an action means using a tool
# Adding a tool to allow the agent to do math
r.add_from_mcp_server(server="uvx mcp-server-calculator")
# Adding a tool to get the current time
r.add_from_mcp_server(server="uvx mcp-timeserver") # Note: you can also add URLs
# Adding a tool to get the current weather
r.add_action(get_weather_info)
# Adding a tool to let the agent stop execution
r.add_action(stop_execution)
async def execute_task(llm, task: str) -> str:
interactions = []
while True:
action = await llm.GetNextAction(task, interactions)
if result := is_result_available(action):
return result
result = r.run(action)
interactions.append(new_interaction(action, result))
llm = LLMClient("gpt-4.1-nano")
task = r.execute_task(llm, "State the current date along with avg temp between LA, NY, and Chicago in Fahrenheit.")
To try it yourself, check out the notebook Simple Agent Demonstration (notebooks/05_simple_agent_demo.ipynb).
Running the Notebooks
To run code from the notebooks/
folder, you'll first need to:
- Install the
uv
python package manager. - Install all dependencies:
uv sync --dev
- Generate necessary BAML code:
uv run baml-cli generate
- Alternatively, you can use the VSCode extension to do it automatically every time you edit a
.baml
file.
- Alternatively, you can use the VSCode extension to do it automatically every time you edit a
相关推荐
Text your favorite pet, after answering 10 questions about their everyday lives!
Carbon footprint calculations breakdown and advices on how to reduce it
You're in a stone cell – can you get out? A classic choose-your-adventure interactive fiction game, based on a meticulously-crafted playbook. With a medieval fantasy setting, infinite choices and outcomes, and dice!
Evaluates language quality of texts, responds with a numerical score between 50-150.
Discover A More Robust Business: Craft tailored value proposition statements, develop a comprehensive business model canvas, conduct detailed PESTLE analysis, and gain strategic insights on enhancing business model elements like scalability, cost structure, and market competition strategies. (v1.18)
Best-in-class AI domain names scoring engine and availability checker. Brandability, domain worth, root keywords and more.
Structured recipes for food and desserts – ingredient lists and step-by-step recipe instructions from any input. Become surprised, try something new or breathe life into grandma's old recipe notebook.
🧑🚀 全世界最好的 llm 资料总结(数据处理、模型训练、模型部署、 O1 模型、 MCP 、小语言模型、视觉语言模型) | Zusammenfassung der weltbesten LLM -Ressourcen.
🔥 1Panel bietet eine intuitive Weboberfläche und einen MCP -Server, um Websites, Dateien, Container, Datenbanken und LLMs auf einem Linux -Server zu verwalten.
Dieses Repository dient zur Entwicklung des Azure MCP -Servers, wodurch Ihre Agenten die Leistung von Azure verleiht.
Dieses Projekt wurde erstellt, um zu demonstrieren, wie wir uns mit verschiedenen Modellkontextprotokollen (MCPs) verbinden können.
⛓️Rugele ist ein leichter, leistungsstarker, leistungsstarker, eingebetteter Komponenten-Orchestrierungsregel-Motor-Rahmen für GO.
PDF wissenschaftliche Papierübersetzung mit erhaltenen Formaten - 基于 ai 完整保留排版的 pdf 文档全文双语翻译 , 支持 支持 支持 支持 google/deeptl/ollama/openai 等服务 提供 cli/gui/mcp/docker/zotero
Reviews
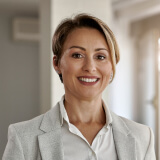
user_wWUrXhUO
As a dedicated user of MCP applications, I have found baml-agents by Elijas to be incredibly efficient and user-friendly. This tool greatly enhances my productivity and streamlines my workflow. The intuitive design and robust functionality ensure that I can achieve my tasks with ease. Highly recommend for anyone in need of a reliable agent tool.