Confidential guide on numerology and astrology, based of GG33 Public information
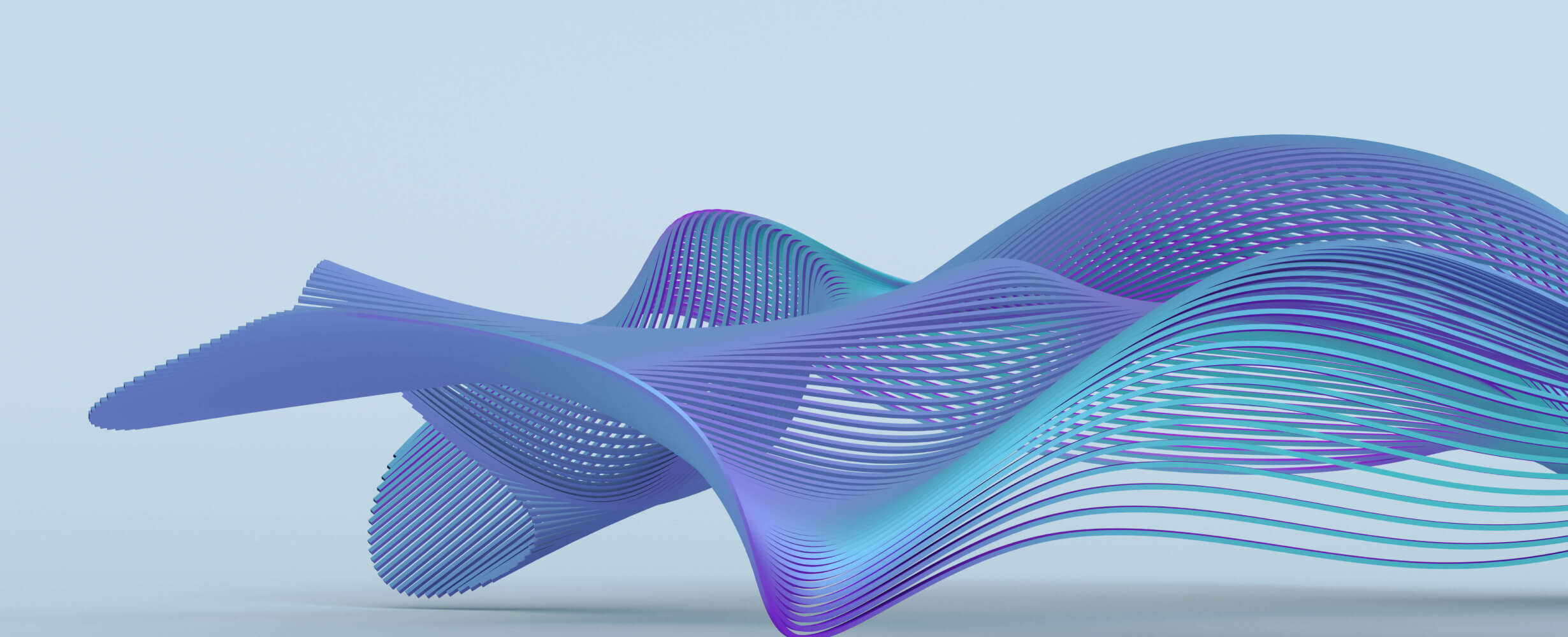
Rag-MCP-Pipeline-Research
Un référentiel d'apprentissage explorant l'intégration du serveur de génération (RAG) et de traitement multi-cloud (MCP) à l'aide de modèles gratuits et open source.
3 years
Works with Finder
1
Github Watches
0
Github Forks
0
Github Stars
RAG-MCP Pipeline Research
A comprehensive research project exploring Retrieval-Augmented Generation (RAG) and Multi-Cloud Processing (MCP) server integration using free and open-source models.
Project Overview
This repository serves as a structured learning and research path for understanding how to integrate Large Language Models (LLMs) with external services through MCP servers, with a focus on practical business applications such as accounting software integration (e.g., QuickBooks).
🌟 Key Features
- No paid API keys required - uses free Hugging Face models
- Run everything locally without external dependencies
- Comprehensive step-by-step documentation for beginners
- Practical examples with working code
Research Modules
Module 0: Prerequisites
Establish a solid foundation before diving into specific areas:
- Programming & Tools: Python, Git/GitHub, Docker
- Basic Concepts: Machine learning, RESTful APIs, cloud services
- AI & LLM Foundations: Understanding transformers, RAG, and prompt engineering
- Development environment setup with free models
Module 1: AI Modeling & LLM Integration
- Understanding different LLM architectures and capabilities
- Integration methods with various LLM providers (Hugging Face, open-source models)
- Fine-tuning strategies for domain-specific tasks
- Evaluation metrics and performance optimization
Module 2: Hosting & Deployment Strategies for AI
- Scalable infrastructure for AI applications
- Cost optimization techniques
- Model serving options (serverless, container-based, dedicated instances)
- Monitoring and observability for LLM applications
Module 3: Deep Dive into MCP Servers
- Architecture and components of MCP servers
- Building secure API gateways for external service integration
- Authentication and authorization patterns
- Command execution protocols and standardization
Module 4: API Integration & Command Execution
- Integration with business software APIs (QuickBooks, etc.)
- Data transformation and normalization
- Error handling and resilience strategies
- Testing and validation methodologies
Module 5: RAG (Retrieval Augmented Generation) & Alternative Strategies
- Vector database selection and optimization
- Document processing pipelines
- Hybrid retrieval approaches
- Alternative augmentation strategies for LLMs
Project Goals
- Gain comprehensive understanding of RAG and MCP server concepts
- Build prototype integrations with popular business software
- Develop a framework for AI-powered data entry and processing
- Create documentation and best practices for future implementations
Getting Started
-
Clone this repository to your local machine
git clone https://github.com/your-username/rag-mcp-pipeline-research.git cd rag-mcp-pipeline-research
-
Run the setup script to prepare your environment
# Navigate to the project directory python src/setup_environment.py
-
Activate the virtual environment
# On Windows venv\Scripts\activate # On macOS/Linux source venv/bin/activate
-
Start with Module 0: Prerequisites
-
Progress through each module sequentially
-
Complete the practical exercises in each section
Why Free Models?
This project intentionally uses free, open-source models from Hugging Face instead of commercial APIs like OpenAI for several reasons:
- Accessibility - Anyone can follow along without financial barriers
- Educational Value - Better understanding of how models work internally
- Privacy - All processing happens locally on your machine
- Flexibility - Easier to customize and fine-tune models for specific needs
- Future-Proofing - Skills transfer to any model, not tied to specific providers
For production applications, you may choose to use commercial APIs for better performance, but the concepts learned here apply universally.
License
MIT
相关推荐
Advanced software engineer GPT that excels through nailing the basics.
I find academic articles and books for research and literature reviews.
Take an adjectivised noun, and create images making it progressively more adjective!
Embark on a thrilling diplomatic quest across a galaxy on the brink of war. Navigate complex politics and alien cultures to forge peace and avert catastrophe in this immersive interstellar adventure.
Découvrez la collection la plus complète et la plus à jour de serveurs MCP sur le marché. Ce référentiel sert de centre centralisé, offrant un vaste catalogue de serveurs MCP open-source et propriétaires, avec des fonctionnalités, des liens de documentation et des contributeurs.
Manipulation basée sur Micropython I2C de l'exposition GPIO de la série MCP, dérivée d'Adafruit_MCP230XX
La communauté du curseur et de la planche à voile, recherchez des règles et des MCP
Un puissant plugin Neovim pour gérer les serveurs MCP (Protocole de contexte modèle)
MCP Server pour récupérer le contenu de la page Web à l'aide du navigateur sans tête du dramwright.
Pont entre les serveurs Olllama et MCP, permettant aux LLM locaux d'utiliser des outils de protocole de contexte de modèle
Un bot de chat IA pour les petites et moyennes équipes, soutenant des modèles tels que Deepseek, Open AI, Claude et Gemini. 专为中小团队设计的 Ai 聊天应用 , 支持 Deepseek 、 Open Ai 、 Claude 、 Gemini 等模型。
🔍 Permettre aux assistants d'IA de rechercher et d'accéder aux informations du package PYPI via une interface MCP simple.
Reviews
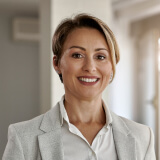
user_CzUsYZ4V
I've been using rag-mcp-pipeline-research by dzikrisyairozi and it's been a game-changer for my projects. The pipeline is robust, easy to implement, and the documentation is very clear. It efficiently handles multi-component processes, making my research workflow seamless. Highly recommend checking it out! https://github.com/dzikrisyairozi/rag-mcp-pipeline-research