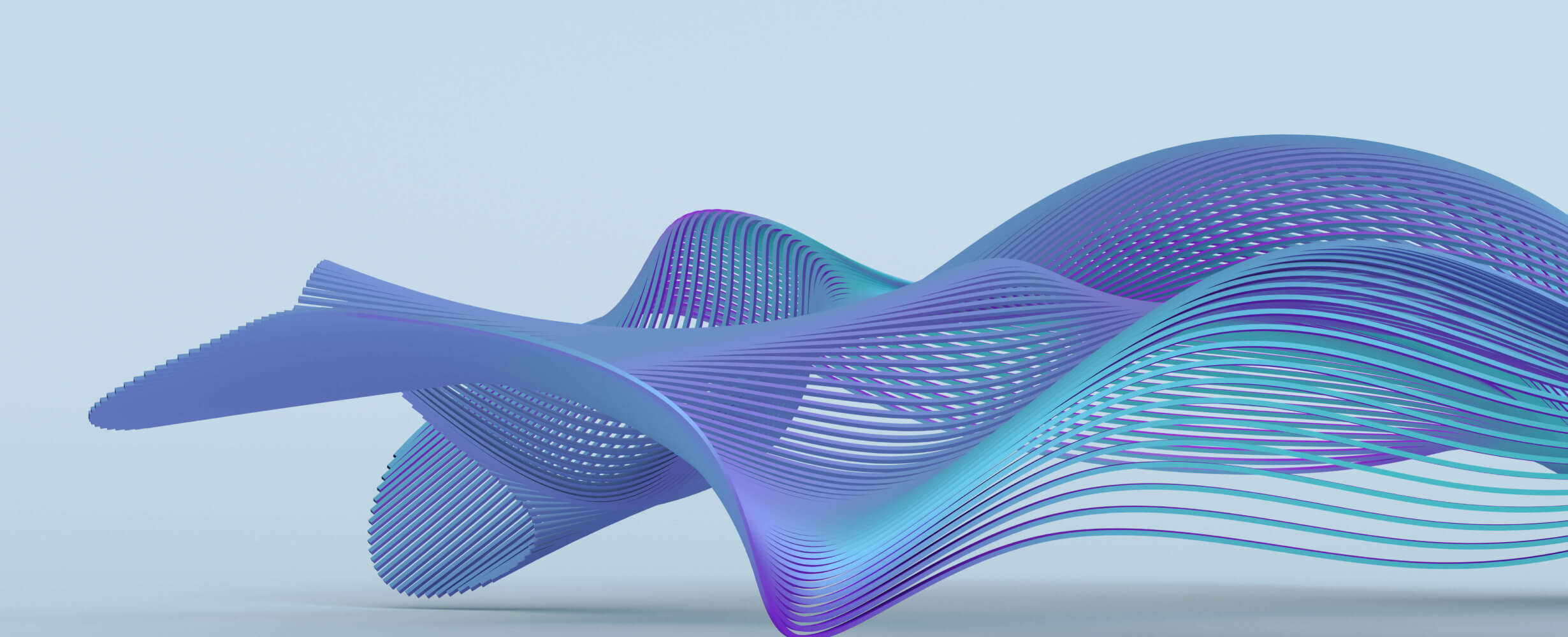
MCP
Multiple-Boundary Clustering and Prioritization
1
Github Watches
3
Github Forks
8
Github Stars
Multiple-Boundary Clustering and Prioritization to Promote Neural Network Retraining
This repository stores our experimental codes and part of the simulated datasets for paper `Multiple-Boundary Clustering and Prioritization to Promote Neural Network Retraining'. MCP is short for our proposed sampling method Multiple-Boundary Clustering and Prioritization.
Dataset:
Part of the datasets lies in the folder 'dataset'. Because some of our simulated test datasets exceed GitHub's file size limit of 100.00 MB, we can only upload part of our datasets in file '/mnist'.
The operational details of simulated test datasets are listed in the README under the folder 'dataset'.
Main experimental codes
You can easily implement our method and 5 baseline methods by yourself or modifying this code.
The main experimental codes are samedist_mnist_retrain.py, samedist_cifar_retrain.py, samedist_svhn_retrain.py. You can modify the list variables 'baselines'(methods) and 'operators'(simulated dataset) to run what you prefer.
baselines =['MCP','LSA','DSA','CES','AAL','SRS']
operators =['fgsm','jsma','bim-a','bim-b','cw-l2','scale','rotation','translation','shear','brightness','contrast']
Baseline methods
MCP. Our method "MCP" is implemented in the "samedist_***_retrain.py" as the function "select_my_optimize".
LSA/DSA. You can directly invoke the functions "fetch_lsa" and "fetch_dsa" from "/LSA_DSA/sa.py" which is downloaded online from the paper "Guiding Deep Learning System Testing Using Surprise Adequacy". These functions can help you get the SA value of each input. The higher the value of SA is, the corresponding test case is more surprise to the DNN under testing. So we select the subset of test cases with higher corresponding SAs.
CES. You can directly invoke the functions "conditional_sample" from "CES/condition.py" which is downloaded online from the git. The original codes have no external interface. In order to call these codes conveniently, we rewrite an interface function, but the internal code and logic about sampling completely reuse their code.
AAL. This approach is written by Matlab. We have write a individual README in the file "AAL" along with all the codes and our experimental setups and results. For short, you can run MATLAB programs and get the intermediate results stored in mnist_finalResults, cifar_finalResults, svhn_finalResults. Then you can run it in our python codes just as the other baseline methods.
SRS. This method is implemented in the "samedist_***_retrain.py" as the function "select_rondom".
相关推荐
I find academic articles and books for research and literature reviews.
Confidential guide on numerology and astrology, based of GG33 Public information
Converts Figma frames into front-end code for various mobile frameworks.
Advanced software engineer GPT that excels through nailing the basics.
Take an adjectivised noun, and create images making it progressively more adjective!
Embark on a thrilling diplomatic quest across a galaxy on the brink of war. Navigate complex politics and alien cultures to forge peace and avert catastrophe in this immersive interstellar adventure.
Discover the most comprehensive and up-to-date collection of MCP servers in the market. This repository serves as a centralized hub, offering an extensive catalog of open-source and proprietary MCP servers, complete with features, documentation links, and contributors.
Micropython I2C-based manipulation of the MCP series GPIO expander, derived from Adafruit_MCP230xx
The all-in-one Desktop & Docker AI application with built-in RAG, AI agents, No-code agent builder, MCP compatibility, and more.
Bridge between Ollama and MCP servers, enabling local LLMs to use Model Context Protocol tools
🧑🚀 全世界最好的LLM资料总结(Agent框架、辅助编程、数据处理、模型训练、模型推理、o1 模型、MCP、小语言模型、视觉语言模型) | Summary of the world's best LLM resources.
Fair-code workflow automation platform with native AI capabilities. Combine visual building with custom code, self-host or cloud, 400+ integrations.
Reviews
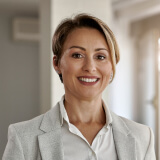
user_f425oVz8
As a dedicated user of MCP, I must say it's an incredibly useful tool developed by actionabletest. Its seamless integration and user-friendly interface make it a joy to use. From navigating the start URL to accessing comprehensive documentation on GitHub, MCP offers a robust experience that caters to both beginners and advanced users. It's a must-have for anyone seeking efficiency and effectiveness in their applications. Highly recommended!