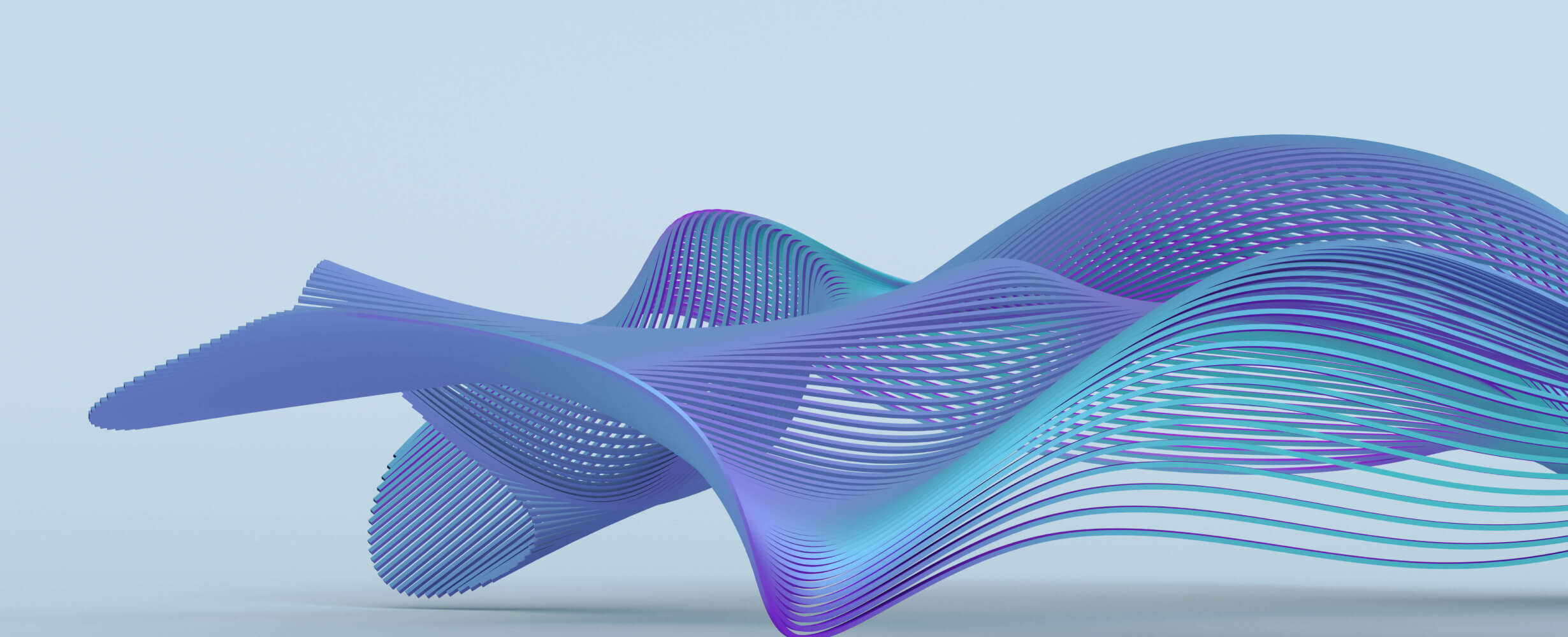
MCP-SSE-Client-Python
Einfacher MCP -Client für Remote -MCP -Server 🌐
1
Github Watches
0
Github Forks
6
Github Stars
MCP SSE Client Python
A Python client for interacting with Model Context Protocol (MCP) endpoints using Server-Sent Events (SSE).
Quick Start
Get up and running in minutes:
# Clone the repository
git clone https://github.com/zanetworker/mcp-sse-client-python.git
cd mcp-sse-client-python
# Install the package
pip install -e .
# Try the interactive Streamlit app
cd mcp-streamlit-app
pip install -r requirements.txt
streamlit run app.py
What is MCP SSE Client?
This client provides a simple interface for:
- Connecting to MCP endpoints via Server-Sent Events
- Discovering and invoking tools with parameters
- Integrating with LLMs (OpenAI, Anthropic, Ollama) for AI-driven tool selection
- Testing tools interactively through a Streamlit UI
Core Features
1. Simple MCP Client
Easily connect to any MCP endpoint and interact with available tools:
import asyncio
from mcp_sse_client import MCPClient
async def main():
# Connect to an MCP endpoint
client = MCPClient("http://localhost:8000/sse")
# List available tools
tools = await client.list_tools()
print(f"Found {len(tools)} tools")
# Invoke a calculator tool
result = await client.invoke_tool(
"calculator",
{"x": 10, "y": 5, "operation": "add"}
)
print(f"Result: {result.content}") # Output: Result: 15
asyncio.run(main())
2. LLM-Powered Tool Selection
Let AI choose the right tool based on natural language queries:
import os
from mcp_sse_client import MCPClient, OpenAIBridge
# Connect to MCP endpoint and create an LLM bridge
client = MCPClient("http://localhost:8000/sse")
bridge = OpenAIBridge(
client,
api_key=os.environ.get("OPENAI_API_KEY"),
model="gpt-4o"
)
# Process a natural language query
result = await bridge.process_query(
"Convert this PDF to text: https://example.com/document.pdf"
)
# The LLM automatically selects the appropriate tool and parameters
if result["tool_call"]:
print(f"Tool: {result['tool_call']['name']}")
print(f"Result: {result['tool_result'].content}")
3. Command-Line Interface
The package includes a powerful CLI tool for interactive testing and analysis:
# Run the CLI tool
python -m mcp_sse_client.examples.llm_example --provider openai --endpoint http://localhost:8000/sse
Configuration Options:
usage: llm_example.py [-h] [--provider {openai,anthropic,ollama}]
[--openai-model {gpt-4o,gpt-4-turbo,gpt-4,gpt-3.5-turbo}]
[--anthropic-model {claude-3-opus-20240229,claude-3-sonnet-20240229,claude-3-haiku-20240307}]
[--ollama-model OLLAMA_MODEL] [--ollama-host OLLAMA_HOST]
[--endpoint ENDPOINT] [--openai-key OPENAI_KEY]
[--anthropic-key ANTHROPIC_KEY]
Example Output:
Starting MCP-LLM Integration Example...
Connecting to MCP server at: http://localhost:8000/sse
Using OpenAI LLM bridge with model: gpt-4o
Fetching tools from server...
=== Available Tools Summary ===
3 tools available:
1. calculator: Perform basic arithmetic operations
Required parameters:
- x (number): First operand
- y (number): Second operand
- operation (string): Operation to perform (add, subtract, multiply, divide)
2. weather: Get current weather for a location
Required parameters:
- location (string): City or location name
3. convert_document: Convert a document to text
Required parameters:
- source (string): URL or file path to the document
Optional parameters:
- enable_ocr (boolean): Whether to use OCR for scanned documents
Entering interactive mode. Type 'quit' to exit.
Enter your query: What's the weather in Berlin?
=== User Query ===
What's the weather in Berlin?
Processing query...
=== LLM Reasoning ===
I need to check the weather in Berlin. Looking at the available tools, there's a "weather" tool that can get the current weather for a location. I'll use this tool with "Berlin" as the location parameter.
=== Tool Selection Decision ===
Selected: weather
Description: Get current weather for a location
Parameters provided:
- location (string, required): Berlin
Description: City or location name
Query to Tool Mapping:
Query: "What's the weather in Berlin?"
Tool: weather
Key parameters: location
=== Tool Execution Result ===
Success: True
Content: {"temperature": 18.5, "conditions": "Partly cloudy", "humidity": 65, "wind_speed": 12}
4. Interactive Testing UI
The included Streamlit app provides a user-friendly interface for:
- Connecting to any MCP endpoint
- Selecting between OpenAI, Anthropic, or Ollama LLMs
- Viewing available tools and their parameters
- Testing tools through natural language in a chat interface
- Visualizing tool selection reasoning and results
To run the Streamlit app:
cd mcp-streamlit-app
streamlit run app.py
Installation
From Source
git clone https://github.com/zanetworker/mcp-sse-client-python.git
cd mcp-sse-client-python
pip install -e .
Using pip (once published)
pip install mcp-sse-client
Supported LLM Providers
The client supports multiple LLM providers for AI-driven tool selection:
- OpenAI: GPT-4o, GPT-4, GPT-3.5-Turbo
- Anthropic: Claude 3 Opus, Claude 3 Sonnet, Claude 3 Haiku
- Ollama: Llama 3, Mistral, and other locally hosted models
API Reference
MCPClient
client = MCPClient(endpoint)
-
endpoint
: The MCP endpoint URL (must be http or https)
Methods
async list_tools() -> List[ToolDef]
Lists available tools from the MCP endpoint.
async invoke_tool(tool_name: str, kwargs: Dict[str, Any]) -> ToolInvocationResult
Invokes a specific tool with parameters.
LLM Bridges
OpenAIBridge
bridge = OpenAIBridge(mcp_client, api_key, model="gpt-4o")
AnthropicBridge
bridge = AnthropicBridge(mcp_client, api_key, model="claude-3-opus-20240229")
OllamaBridge
bridge = OllamaBridge(mcp_client, model="llama3", host=None)
Common Bridge Methods
async process_query(query: str) -> Dict[str, Any]
Processes a user query through the LLM and executes any tool calls.
Requirements
- Python 3.7+
-
requests
-
sseclient-py
-
pydantic
-
openai
(for OpenAI integration) -
anthropic
(for Anthropic integration) -
ollama
(for Ollama integration) -
streamlit
(for the interactive test app)
Development
For information on development setup, contributing guidelines, and available make commands, see DEVELOPMENT.md.
License
This project is licensed under the MIT License - see the LICENSE file for details.
相关推荐
I find academic articles and books for research and literature reviews.
Confidential guide on numerology and astrology, based of GG33 Public information
Converts Figma frames into front-end code for various mobile frameworks.
Advanced software engineer GPT that excels through nailing the basics.
Take an adjectivised noun, and create images making it progressively more adjective!
Embark on a thrilling diplomatic quest across a galaxy on the brink of war. Navigate complex politics and alien cultures to forge peace and avert catastrophe in this immersive interstellar adventure.
Entdecken Sie die umfassendste und aktuellste Sammlung von MCP-Servern auf dem Markt. Dieses Repository dient als zentraler Hub und bietet einen umfangreichen Katalog von Open-Source- und Proprietary MCP-Servern mit Funktionen, Dokumentationslinks und Mitwirkenden.
Die All-in-One-Desktop & Docker-AI-Anwendung mit integriertem Lappen, AI-Agenten, No-Code-Agent Builder, MCP-Kompatibilität und vielem mehr.
Ein leistungsstarkes Neovim -Plugin für die Verwaltung von MCP -Servern (Modellkontextprotokoll)
MCP -Server für den Fetch -Webseiteninhalt mit dem Headless -Browser von Dramatikern.
Brücke zwischen Ollama und MCP -Servern und ermöglicht es lokalen LLMs, Modellkontextprotokoll -Tools zu verwenden
Fair-Code-Workflow-Automatisierungsplattform mit nativen KI-Funktionen. Kombinieren Sie visuelles Gebäude mit benutzerdefiniertem Code, SelbstHost oder Cloud, 400+ Integrationen.
Reviews
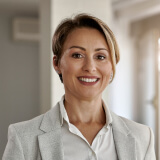
user_pSL30yZy
I've been using the mcp-sse-client-python by zanetworker and it has proven to be an invaluable tool for managing server-sent events in Python. It offers seamless integration and is very user-friendly. The comprehensive documentation on the GitHub page makes setup and usage straightforward. Highly recommended for anyone needing a reliable SSE client in their Python projects! Check it out at https://github.com/zanetworker/mcp-sse-client-python.