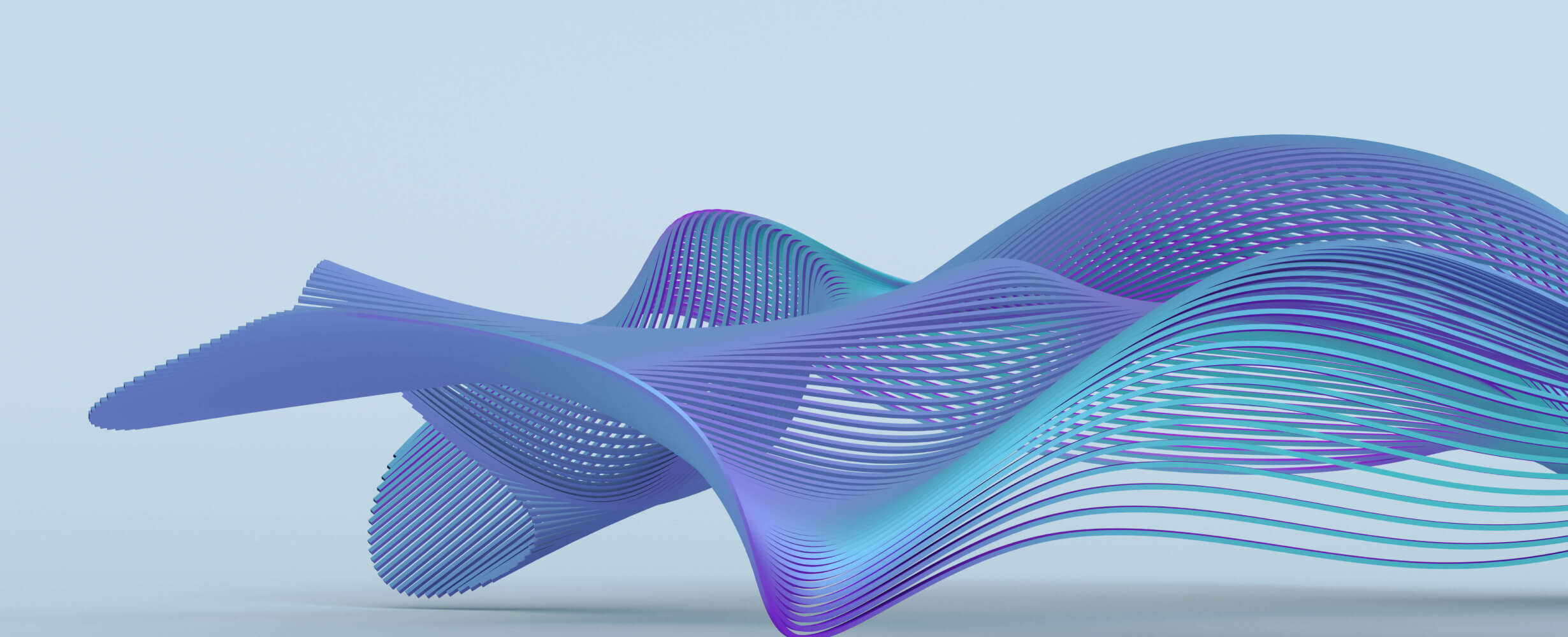
MCP-Titan-Cognitive Memory
1
Github Watches
11
Github Forks
57
Github Stars
🧠 MCP - Titan Memory Server implementation
Colaboration between @jasonkneen and @ExpressionsBot
Follow us on X
An implementation inspired by Google Research's paper "Generative AI for Programming: A Common Task Framework". This server provides a neural memory system that can learn and predict sequences while maintaining state through a memory vector, following principles outlined in the research for improved code generation and understanding.
📚 Research Background
This implementation draws from the concepts presented in the Google Research paper (Muennighoff et al., 2024) which introduces a framework for evaluating and improving code generation models. The Titan Memory Server implements key concepts from the paper:
- Memory-augmented sequence learning
- Surprise metric for novelty detection
- Manifold optimization for stable learning
- State maintenance through memory vectors
These features align with the paper's goals of improving code understanding and generation through better memory and state management.
🚀 Features
- Neural memory model with configurable dimensions
- Sequence learning and prediction
- Surprise metric calculation
- Model persistence (save/load)
- Memory state management
- Full MCP tool integration
📦 Installation
# Install dependencies
npm install
# Build the project
npm run build
# Run tests
npm test
🛠️ Available MCP Tools
1. 🎯 init_model
Initialize the Titan Memory model with custom configuration.
{
inputDim?: number; // Input dimension (default: 64)
outputDim?: number; // Output/Memory dimension (default: 64)
}
2. 📚 train_step
Perform a single training step with current and next state vectors.
{
x_t: number[]; // Current state vector
x_next: number[]; // Next state vector
}
3. 🔄 forward_pass
Run a forward pass through the model with an input vector.
{
x: number[]; // Input vector
}
4. 💾 save_model
Save the model to a specified path.
{
path: string; // Path to save the model
}
5. 📂 load_model
Load the model from a specified path.
{
path: string; // Path to load the model from
}
6. ℹ️ get_status
Get current model status and configuration.
{} // No parameters required
7. 🔄 train_sequence
Train the model on a sequence of vectors.
{
sequence: number[][]; // Array of vectors to train on
}
🌟 Example Usage
// Initialize model
await callTool('init_model', { inputDim: 64, outputDim: 64 });
// Train on a sequence
const sequence = [
[1, 0, 0, /* ... */],
[0, 1, 0, /* ... */],
[0, 0, 1, /* ... */]
];
await callTool('train_sequence', { sequence });
// Run forward pass
const result = await callTool('forward_pass', {
x: [1, 0, 0, /* ... */]
});
🔧 Technical Details
- Built with TensorFlow.js for efficient tensor operations
- Uses manifold optimization for stable learning
- Implements surprise metric for novelty detection
- Memory management with proper tensor cleanup
- Type-safe implementation with TypeScript
- Comprehensive error handling
🧪 Testing
The project includes comprehensive tests covering:
- Model initialization and configuration
- Training and forward pass operations
- Memory state management
- Model persistence
- Edge cases and error handling
- Tensor cleanup and memory management
Run tests with:
npm test
🔍 Implementation Notes
- All tensor operations are wrapped in
tf.tidy()
for proper memory management - Implements proper error handling with detailed error messages
- Uses type-safe MCP tool definitions
- Maintains memory state between operations
- Handles floating-point precision issues with epsilon tolerance
📝 License
MIT License - feel free to use and modify as needed!
相关推荐
I find academic articles and books for research and literature reviews.
Confidential guide on numerology and astrology, based of GG33 Public information
Converts Figma frames into front-end code for various mobile frameworks.
Advanced software engineer GPT that excels through nailing the basics.
Take an adjectivised noun, and create images making it progressively more adjective!
Emulating Dr. Jordan B. Peterson's style in providing life advice and insights.
Embark on a thrilling diplomatic quest across a galaxy on the brink of war. Navigate complex politics and alien cultures to forge peace and avert catastrophe in this immersive interstellar adventure.
Entdecken Sie die umfassendste und aktuellste Sammlung von MCP-Servern auf dem Markt. Dieses Repository dient als zentraler Hub und bietet einen umfangreichen Katalog von Open-Source- und Proprietary MCP-Servern mit Funktionen, Dokumentationslinks und Mitwirkenden.
Die All-in-One-Desktop & Docker-AI-Anwendung mit integriertem Lappen, AI-Agenten, No-Code-Agent Builder, MCP-Kompatibilität und vielem mehr.
Ein leistungsstarkes Neovim -Plugin für die Verwaltung von MCP -Servern (Modellkontextprotokoll)
MCP -Server für den Fetch -Webseiteninhalt mit dem Headless -Browser von Dramatikern.
Brücke zwischen Ollama und MCP -Servern und ermöglicht es lokalen LLMs, Modellkontextprotokoll -Tools zu verwenden
Fair-Code-Workflow-Automatisierungsplattform mit nativen KI-Funktionen. Kombinieren Sie visuelles Gebäude mit benutzerdefiniertem Code, SelbstHost oder Cloud, 400+ Integrationen.
🧑🚀 全世界最好的 llm 资料总结(数据处理、模型训练、模型部署、 O1 模型、 MCP 、小语言模型、视觉语言模型) | Zusammenfassung der weltbesten LLM -Ressourcen.
Reviews
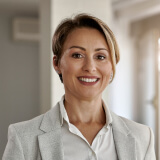
user_xHHE25AY
The mcp-titan-cognitive-memory by Synthience is an exceptional tool for enhancing cognitive processes. Its stellar design and seamless integration have significantly boosted my memory and productivity. The user-friendly interface and comprehensive documentation make it accessible for both beginners and advanced users. Highly recommend checking it out!