Confidential guide on numerology and astrology, based of GG33 Public information
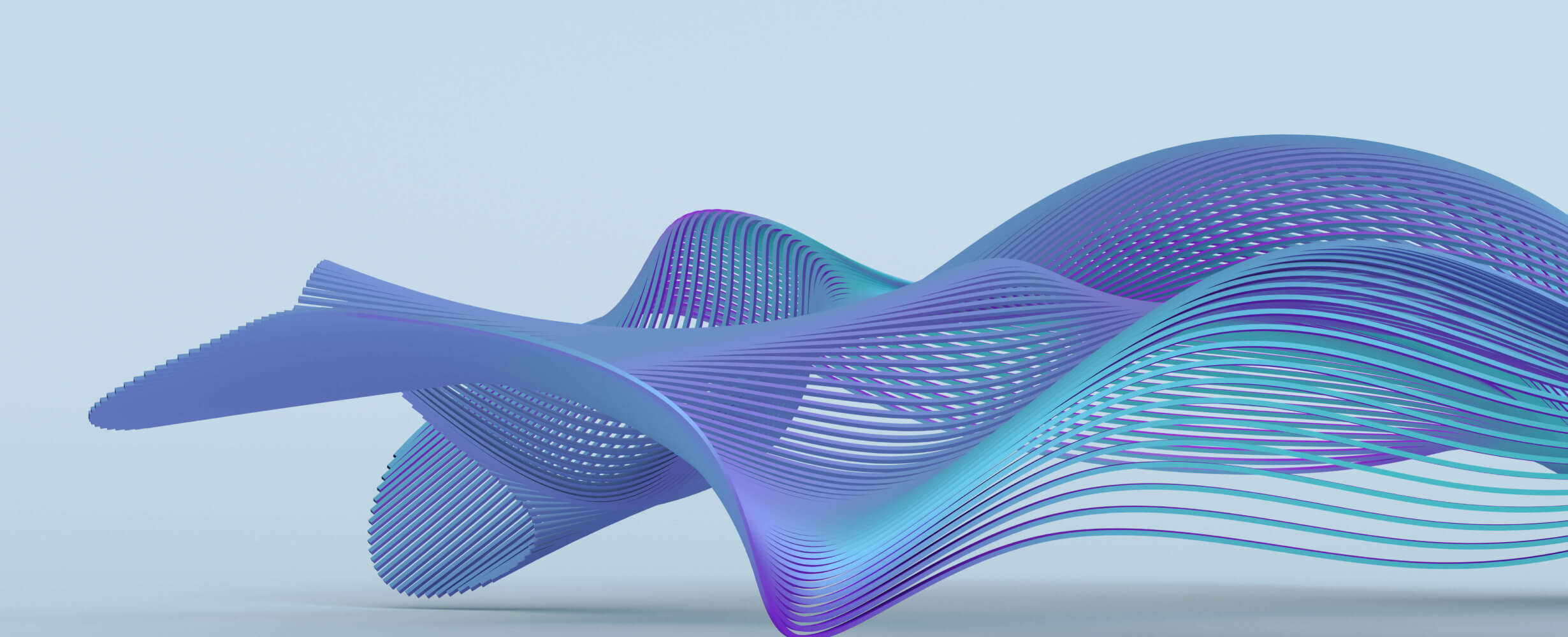
2018aicity_mcprl
3 years
Works with Finder
3
Github Watches
14
Github Forks
26
Github Stars
Unsupervised Anomaly Detection for Traffic Surveillance Based on Background Modeling
This work participates in Nvidia AI City Challenge CVPR 2018 Workshop.
This repository contains our source code of Track-2 in the NVIDIA AI City Challenge at CVPR 2018 Workshop.
Introduction
The pipeline of our system is as follow:
As shown in the picture, we get the background frames from the original frames. Then we detect vehicles in background images using Faster-RCNN. After that, to eliminate false detected bounding boxes, we utilize VGG as a powerful classifier. We determine anomalous vehicles based on the bounding boxes produced by the former module. When meeting camera movement or vehicles waiting for red lights, we will compare the similarity between two candidates with the help of ResNet50 trained with triplet loss. For every detected abnormal vehicles, we go back to find the accurate timestamp when the abnormal happens. More details can be found in our paper.
Dependencies
- Python2
- Caffe
- pytorch
- cv2
- PIL
- Numpy
Code structure
Because it takes a lone time to run our system on the entire evaluation dataset, which contains about 1500 minuntes video in total, we split and run our system in three part. What's more, it makes debugging easier. First, we generate the background frames. Next, we detecte vechiles using faster-rcnn in multi-scale, which is follower by a VGG16 classifier. At last, we obtain all anomalies according to saved bounding boxes. If you want, you can also run the whole system end-to-end, but you have to combine the code together. When meeting some trouble, you should feel free to contact me
- Run
python ./extract_background.py
. Then, you will get all original frames and background frames saved in./data/all_imgs/all
and./data/all_imgs/bg
separately, which are both used in the following modules. - The
./classification
contains the code to train classification model. You can also download our model rather than train it yourself. - The
./similar
contains the code to train similarity comparison model. You can also download our model rather than train it yourself. - The
./data
is the directory to save all images and models used in our work. This is some of our data for classification and the source other data are illustruted here. Here you can download all models used by us. - The
./py-faster-rcnn
contains almost all the scripts of our system.
More details can be found in corresponding subfolders.
Reference
JiaYi Wei, JianFei Zhao, YanYun Zhao, ZhiCheng Zhao, "Unsupervised Anomaly Detection for Traffic Surveillance Based on Background Modeling", in Proc. IEEE/CVF Conf. Comput. Vis. Pattern Recogn. Workshops (CVPRW), Jun. 2018.
Contact
If you have any question about our work, you can contact JiaYi Wei
相关推荐
Converts Figma frames into front-end code for various mobile frameworks.
Advanced software engineer GPT that excels through nailing the basics.
Take an adjectivised noun, and create images making it progressively more adjective!
Siri Shortcut Finder – your go-to place for discovering amazing Siri Shortcuts with ease
I find academic articles and books for research and literature reviews.
Entdecken Sie die umfassendste und aktuellste Sammlung von MCP-Servern auf dem Markt. Dieses Repository dient als zentraler Hub und bietet einen umfangreichen Katalog von Open-Source- und Proprietary MCP-Servern mit Funktionen, Dokumentationslinks und Mitwirkenden.
MCP -Server für den Fetch -Webseiteninhalt mit dem Headless -Browser von Dramatikern.
Ein leistungsstarkes Neovim -Plugin für die Verwaltung von MCP -Servern (Modellkontextprotokoll)
Brücke zwischen Ollama und MCP -Servern und ermöglicht es lokalen LLMs, Modellkontextprotokoll -Tools zu verwenden
🧑🚀 全世界最好的 llm 资料总结(数据处理、模型训练、模型部署、 O1 模型、 MCP 、小语言模型、视觉语言模型) | Zusammenfassung der weltbesten LLM -Ressourcen.
Die All-in-One-Desktop & Docker-AI-Anwendung mit integriertem Lappen, AI-Agenten, No-Code-Agent Builder, MCP-Kompatibilität und vielem mehr.
Awesome MCP -Server - eine kuratierte Liste von Modellkontext -Protokollservern für Modellkontext
Reviews
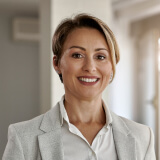
user_z2npzsUt
As a dedicated user of the 2018AICITY_MCPRL, I am thoroughly impressed with its performance and capabilities. Developed by NVIDIAAICITYCHALLENGE, it delivers top-notch results for city challenges, showcasing exceptional precision and innovation. The repository on GitHub is well-organized and the documentation is exceptionally clear, making it easy to integrate and utilize. Highly recommended for professionals looking for a robust machine learning solution!