I craft unique cereal names, stories, and ridiculously cute Cereal Baby images.
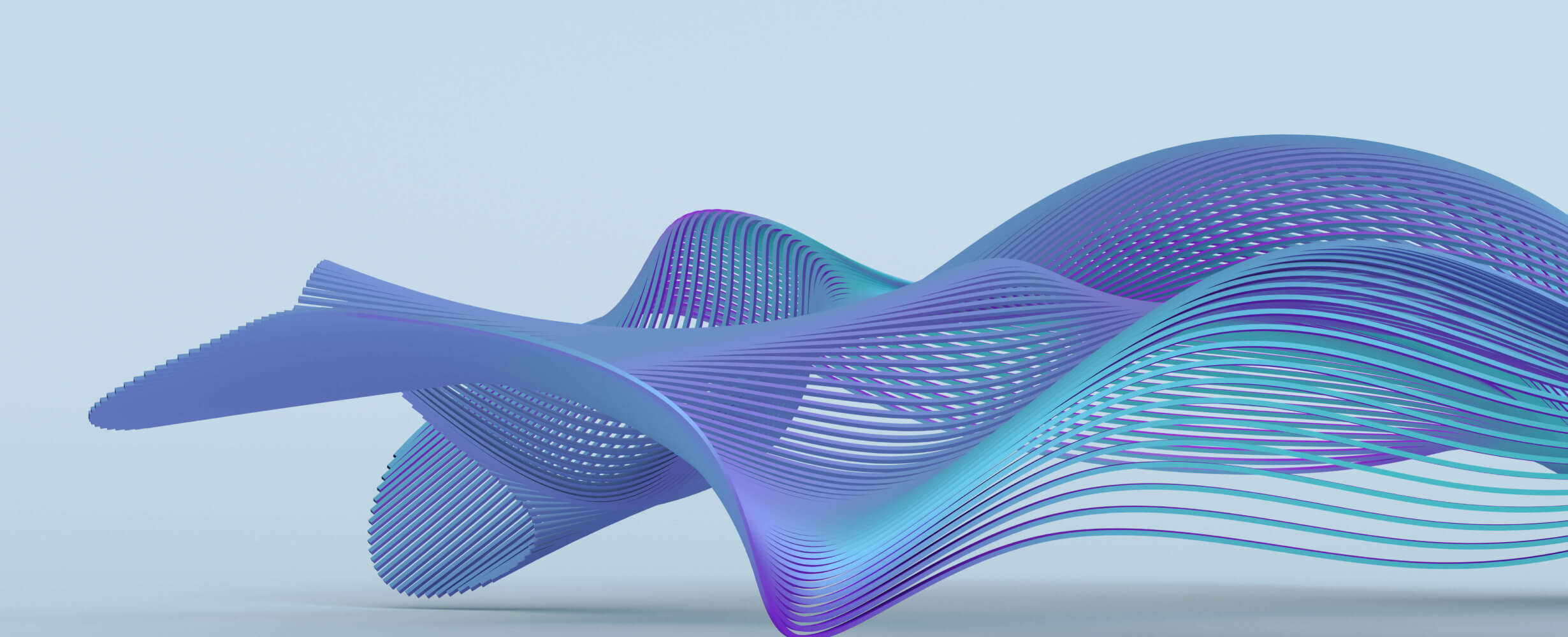
Python-Memory-MCP-Server
1
Github Watches
3
Github Forks
10
Github Stars
Memory MCP Server
A Model Context Protocol (MCP) server that provides knowledge graph functionality for managing entities, relations, and observations in memory, with strict validation rules to maintain data consistency.
Installation
Install the server in Claude Desktop:
mcp install main.py -v MEMORY_FILE_PATH=/path/to/memory.jsonl
Data Validation Rules
Entity Names
- Must start with a lowercase letter
- Can contain lowercase letters, numbers, and hyphens
- Maximum length of 100 characters
- Must be unique within the graph
- Example valid names:
python-project
,meeting-notes-2024
,user-john
Entity Types
The following entity types are supported:
-
person
: Human entities -
concept
: Abstract ideas or principles -
project
: Work initiatives or tasks -
document
: Any form of documentation -
tool
: Software tools or utilities -
organization
: Companies or groups -
location
: Physical or virtual places -
event
: Time-bound occurrences
Observations
- Non-empty strings
- Maximum length of 500 characters
- Must be unique per entity
- Should be factual and objective statements
- Include timestamp when relevant
Relations
The following relation types are supported:
-
knows
: Person to person connection -
contains
: Parent/child relationship -
uses
: Entity utilizing another entity -
created
: Authorship/creation relationship -
belongs-to
: Membership/ownership -
depends-on
: Dependency relationship -
related-to
: Generic relationship
Additional relation rules:
- Both source and target entities must exist
- Self-referential relations not allowed
- No circular dependencies allowed
- Must use predefined relation types
Usage
The server provides tools for managing a knowledge graph:
Get Entity
result = await session.call_tool("get_entity", {
"entity_name": "example"
})
if not result.success:
if result.error_type == "NOT_FOUND":
print(f"Entity not found: {result.error}")
elif result.error_type == "VALIDATION_ERROR":
print(f"Invalid input: {result.error}")
else:
print(f"Error: {result.error}")
else:
entity = result.data
print(f"Found entity: {entity}")
Get Graph
result = await session.call_tool("get_graph", {})
if result.success:
graph = result.data
print(f"Graph data: {graph}")
else:
print(f"Error retrieving graph: {result.error}")
Create Entities
# Valid entity creation
entities = [
Entity(
name="python-project", # Lowercase with hyphens
entityType="project", # Must be a valid type
observations=["Started development on 2024-01-29"]
),
Entity(
name="john-doe",
entityType="person",
observations=["Software engineer", "Joined team in 2024"]
)
]
result = await session.call_tool("create_entities", {
"entities": entities
})
if not result.success:
if result.error_type == "VALIDATION_ERROR":
print(f"Invalid entity data: {result.error}")
else:
print(f"Error creating entities: {result.error}")
Add Observation
# Valid observation
result = await session.call_tool("add_observation", {
"entity": "python-project",
"observation": "Completed initial prototype" # Must be unique for entity
})
if not result.success:
if result.error_type == "NOT_FOUND":
print(f"Entity not found: {result.error}")
elif result.error_type == "VALIDATION_ERROR":
print(f"Invalid observation: {result.error}")
else:
print(f"Error adding observation: {result.error}")
Create Relation
# Valid relation
result = await session.call_tool("create_relation", {
"from_entity": "john-doe",
"to_entity": "python-project",
"relation_type": "created" # Must be a valid type
})
if not result.success:
if result.error_type == "NOT_FOUND":
print(f"Entity not found: {result.error}")
elif result.error_type == "VALIDATION_ERROR":
print(f"Invalid relation data: {result.error}")
else:
print(f"Error creating relation: {result.error}")
Search Memory
result = await session.call_tool("search_memory", {
"query": "most recent workout" # Supports natural language queries
})
if result.success:
if result.error_type == "NO_RESULTS":
print(f"No results found: {result.error}")
else:
results = result.data
print(f"Search results: {results}")
else:
print(f"Error searching memory: {result.error}")
The search functionality supports:
- Temporal queries (e.g., "most recent", "last", "latest")
- Activity queries (e.g., "workout", "exercise")
- General entity searches
- Fuzzy matching with 80% similarity threshold
- Weighted search across:
- Entity names (weight: 1.0)
- Entity types (weight: 0.8)
- Observations (weight: 0.6)
Delete Entities
result = await session.call_tool("delete_entities", {
"names": ["python-project", "john-doe"]
})
if not result.success:
if result.error_type == "NOT_FOUND":
print(f"Entity not found: {result.error}")
else:
print(f"Error deleting entities: {result.error}")
Delete Relation
result = await session.call_tool("delete_relation", {
"from_entity": "john-doe",
"to_entity": "python-project"
})
if not result.success:
if result.error_type == "NOT_FOUND":
print(f"Entity not found: {result.error}")
else:
print(f"Error deleting relation: {result.error}")
Flush Memory
result = await session.call_tool("flush_memory", {})
if not result.success:
print(f"Error flushing memory: {result.error}")
Error Types
The server uses the following error types:
-
NOT_FOUND
: Entity or resource not found -
VALIDATION_ERROR
: Invalid input data -
INTERNAL_ERROR
: Server-side error -
ALREADY_EXISTS
: Resource already exists -
INVALID_RELATION
: Invalid relation between entities
Response Models
All tools return typed responses using these models:
EntityResponse
class EntityResponse(BaseModel):
success: bool
data: Optional[Dict[str, Any]] = None
error: Optional[str] = None
error_type: Optional[str] = None
GraphResponse
class GraphResponse(BaseModel):
success: bool
data: Optional[Dict[str, Any]] = None
error: Optional[str] = None
error_type: Optional[str] = None
OperationResponse
class OperationResponse(BaseModel):
success: bool
error: Optional[str] = None
error_type: Optional[str] = None
Development
Running Tests
pytest tests/
Adding New Features
- Update validation rules in
validation.py
- Add tests in
tests/test_validation.py
- Implement changes in
knowledge_graph_manager.py
相关推荐
Evaluator for marketplace product descriptions, checks for relevancy and keyword stuffing.
This GPT assists in finding a top-rated business CPA - local or virtual. We account for their qualifications, experience, testimonials and reviews. Business operators provide a short description of your business, services wanted, and city or state.
I find academic articles and books for research and literature reviews.
Confidential guide on numerology and astrology, based of GG33 Public information
Découvrez la collection la plus complète et la plus à jour de serveurs MCP sur le marché. Ce référentiel sert de centre centralisé, offrant un vaste catalogue de serveurs MCP open-source et propriétaires, avec des fonctionnalités, des liens de documentation et des contributeurs.
Manipulation basée sur Micropython I2C de l'exposition GPIO de la série MCP, dérivée d'Adafruit_MCP230XX
L'application tout-en-un desktop et Docker AI avec chiffon intégré, agents AI, constructeur d'agent sans code, compatibilité MCP, etc.
Une passerelle API unifiée pour intégrer plusieurs API d'explorateur de blockchain de type étherscan avec la prise en charge du protocole de contexte modèle (MCP) pour les assistants d'IA.
Reviews
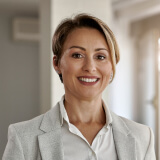
user_LgxwHo0c
As a loyal user of the mcp-agent-server by Leonard907, I must say this product is fantastic! It integrates seamlessly into our existing systems and provides reliable performance consistently. The user interface is intuitive, making it accessible for both technical and non-technical team members. Check it out here: https://mcp.so/server/mcp-agent-server/Leonard907. Highly recommended!