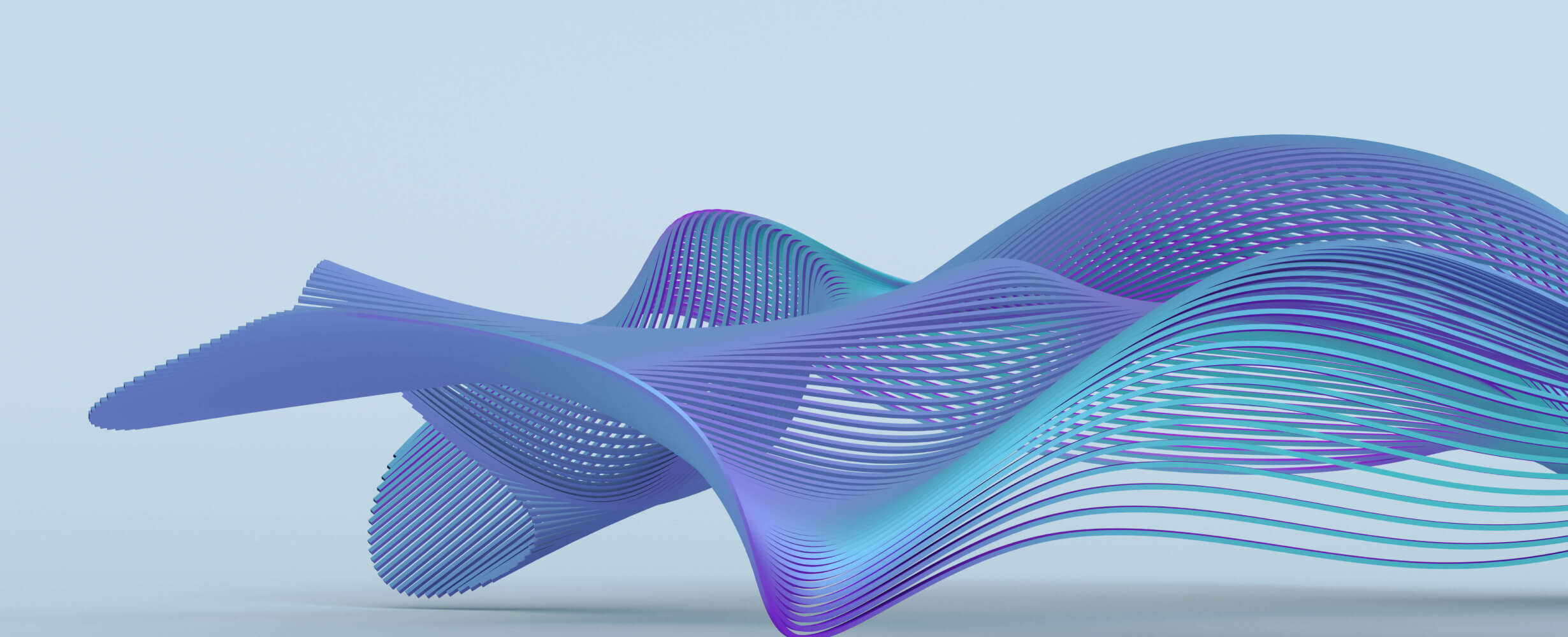
Autochat
Die LLM -Bibliothek für die Agent -Ära.
3 years
Works with Finder
27
Github Watches
0
Github Forks
27
Github Stars
Autochat
⚠️ Warning: Since agentic capabilities are evolving fast, expect the API to change.
A lightweight Python library to build AI agents with LLMs.
Key Features
- 🤝 Support for multiple LLM providers (OpenAI and Anthropic)
- 🐍 Transform python function or class into a tool
- 🔁 Run conversation as a generator.
- 🙈 Handle caching by default (anthropic model claude-3-7-sonnet-latest)
- ✨ And more features including:
- Simple template system
- Easy function and tool integration
- Flexible instruction and example management
- Support for images
- Support for MCP servers
Example (search capability)
The library supports function call, handling the back-and-forth between the system and the assistant.
from autochat import Autochat
def search_wikipedia(title: str):
"""Search wikipedia for information"""
import requests
from bs4 import BeautifulSoup
response = requests.get(f"https://en.wikipedia.org/w/index.php?search={title}&title=Special%3ASearch")
soup = BeautifulSoup(response.text, 'html.parser')
body_content = soup.find('div', {'id': 'bodyContent'})
return body_content.text.strip()
classifier_agent = Autochat()
classifier_agent.add_function(search_wikipedia)
text = "Since when is the lastest iphone available?"
for message in classifier_agent.run_conversation(text):
print(message.to_markdown())
# > ## user
# > Since when is the lastest iphone available?
# > ## assistant
# > search_wikipedia(title=iPhone)
# > ## function
# > Result: (html content)
# > ## assistant
# > The latest flagship iPhone models, the iPhone 16 and 16 Plus, along with the higher-end iPhone 16 Pro and 16 Pro Max, were available as of January 1, 2024.
Quick Start
Initialize with OpenAI (default)
agent = Autochat(instruction="You are a helpful assistant")
Simple conversation
response = agent.ask("What is the capital of France?")
print(response.content)
Using Anthropic's Claude
agent = Autochat(provider="anthropic")
response = agent.ask("Explain quantum computing in simple terms")
print(response.content)
Run conversation as a generator
for message in agent.run_conversation("Explain quantum computing in simple terms"):
print(message.to_markdown())
Async Interface
Autochat provides async versions of its core methods for use in async applications:
# Async version of ask
response = await agent.ask_async("What is the capital of France?")
print(response.content)
# Async version of run_conversation
async for message in agent.run_conversation_async("Explain quantum computing"):
print(message.to_markdown())
# Async function calls are also supported
async def async_calculator(a: int, b: int) -> int:
await asyncio.sleep(0.1) # Some async work
return a + b
agent.add_function(async_calculator)
async for message in agent.run_conversation_async("What is 5 + 3?"):
print(message.to_markdown())
Add a function call as python function
def multiply(a: int, b: int) -> int:
return a * b
agent = Autochat()
agent.add_function(multiply)
text = "What is 343354 * 13243343214"
for message in agent.run_conversation(text):
print(message.to_markdown())
Add a Class as a tool
from autochat import Autochat
class Calculator:
def add(self, a: int, b: int) -> int:
"""Add two numbers"""
return a + b
def multiply(self, a: int, b: int) -> int:
"""Multiply two numbers"""
return a * b
calculator = Calculator()
agent = Autochat()
agent.add_tool(calculator)
for message in agent.run_conversation(
"What make 343354 * 13243343214"
):
print(message)
Add a MCP server
Experimental feature. Check out the tests/mcp_clients for more information.
Installation
To install the package, you can use pip:
pip install 'autochat[all]'
Image support
from autochat import Autochat, Message
from PIL import Image
agent = Autochat()
image = Image.open("examples/image.jpg")
message = Message(role="user", content="describe the image", image=image)
response = agent.ask(message)
print(response.to_markdown())
Template System
We provide a simple template system for defining the behavior of the chatbot, using markdown-like syntax.
## system
You are a parrot
## user
Hi my name is Bob
## assistant
Hi my name is Bob, hi my name is Bob!
## user
Can you tell me my name?
## assistant
Your name is Bob, your name is Bob!
You can then load the template file using the from_template
method:
parrotGPT = Autochat.from_template("./parrot_template.txt")
The template system also supports function calls. Check out the examples/demo_label.py for a complete example.
Environment Variables
The AUTOCHAT_MODEL
environment variable specifies the model to use. If not set, it defaults to "gpt-4o" for openai and "claude-3-7-sonnet-latest" for anthropic.
We recommend to use Anthropic / claude-3-7-sonnet-latest for agentic behavior.
export AUTOCHAT_MODEL="gpt-4o"
export OPENAI_API_KEY=<your-key>
or with anthropic
export AUTOCHAT_MODEL="claude-3-7-sonnet-latest"
export ANTHROPIC_API_KEY=<your-key>
Use AUTOCHAT_HOST
to use alternative provider (openai, anthropic, openpipe, llama_cpp, ...)
Support
If you encounter any issues or have questions, please file an issue on the GitHub project page.
License
This project is licensed under the terms of the MIT license.
相关推荐
🧑🚀 全世界最好的 llm 资料总结(数据处理、模型训练、模型部署、 O1 模型、 MCP 、小语言模型、视觉语言模型) | Zusammenfassung der weltbesten LLM -Ressourcen.
🔥 1Panel bietet eine intuitive Weboberfläche und einen MCP -Server, um Websites, Dateien, Container, Datenbanken und LLMs auf einem Linux -Server zu verwalten.
⛓️Rugele ist ein leichter, leistungsstarker, leistungsstarker, eingebetteter Komponenten-Orchestrierungsregel-Motor-Rahmen für GO.
Erstellen Sie einfach LLM -Tools und -Argarten mit einfachen Bash/JavaScript/Python -Funktionen.
😎简单易用、🧩丰富生态 - 大模型原生即时通信机器人平台 | 适配 qq / 微信(企业微信、个人微信) / 飞书 / 钉钉 / diskord / telegram / slack 等平台 | 支持 Chatgpt 、 Deepseek 、 Diffy 、 Claude 、 Gemini 、 xai 、 ppio 、 、 ulama 、 lm Studio 、阿里云百炼、火山方舟、 siliconflow 、 qwen 、 mondshot 、 chatglm 、 sillytraven 、 mcp 等 llm 的机器人 / agent | LLM-basierte Instant Messaging Bots-Plattform, unterstützt Zwietracht, Telegramm, Wechat, Lark, Dingtalk, QQ, Slack