I craft unique cereal names, stories, and ridiculously cute Cereal Baby images.
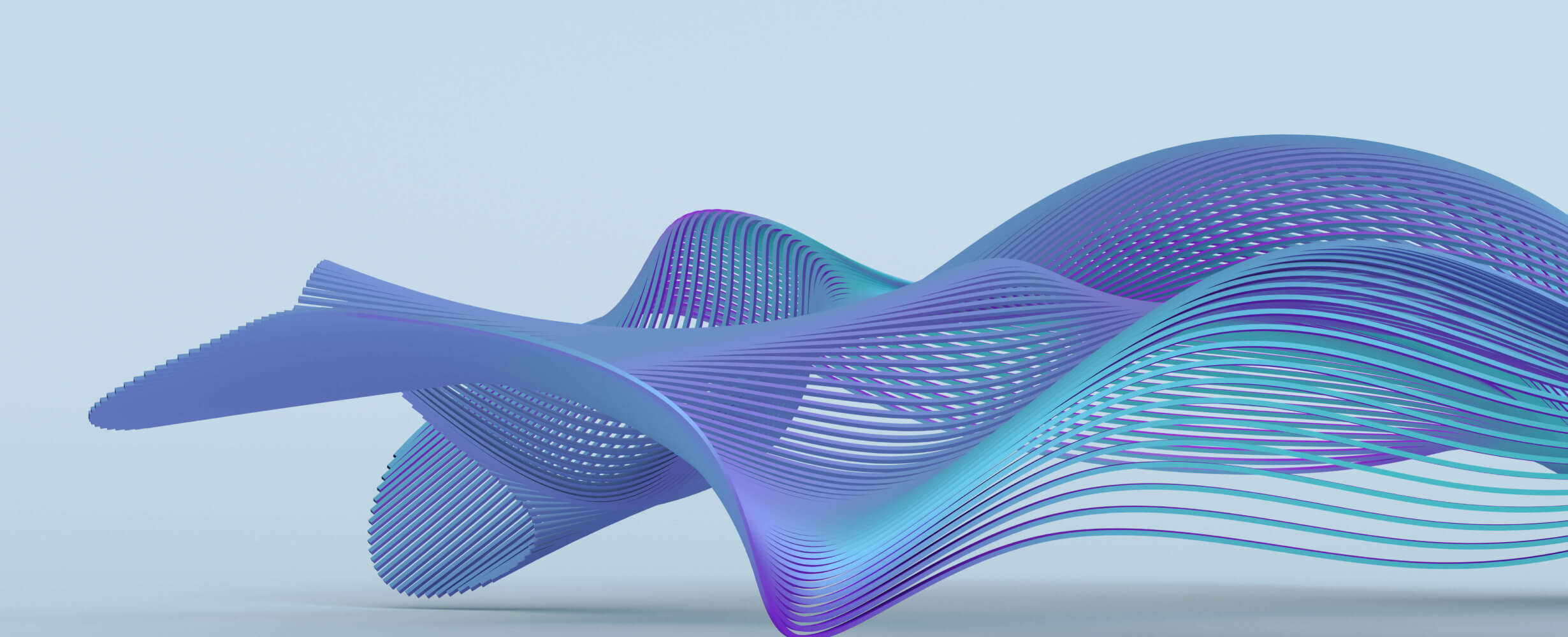
codier_db
Ein intelligentes Codespeichersystem, das Vektoreinbettung, strukturierte Datenbanken und Wissensgrafiken nutzt, um Codemuster mit semantischen Suchfunktionen, Qualitätsmetriken und Beziehungsmodellierung zu speichern, abzurufen und zu analysieren. Entwickelt, um Programmierworkflows durch den kontextuellen Rückruf von Best Practices, Algorithmen und Lösungen zu verbessern.
1
Github Watches
1
Github Forks
2
Github Stars
Coder DB - AI Memory Enhancement System
A structured memory system for AI assistants to enhance coding capabilities using database integration utilizing Claude Desktop and MCP Servers.
Overview
This system leverages multiple database types to create a comprehensive memory system for coding assistance:
- Qdrant Vector Database: For semantic search and retrieval of code patterns
- SQLite Database: For structured algorithm storage and versioning
- Knowledge Graph: For representing relationships between coding concepts
Database Usage Guide
Qdrant Memory Storage
For storing and retrieving code snippets, patterns, and solutions by semantic meaning.
What to store:
- Reusable code patterns with explanations
- Solutions to complex problems
- Best practices and design patterns
- Documentation fragments and explanations
Enhanced Metadata:
- Language and framework details
- Complexity level (simple, intermediate, advanced)
- Dependencies and requirements
- Quality metrics (cyclomatic complexity, documentation coverage)
- User feedback and ratings
Example Usage:
# Storing a code pattern
information = {
"type": "code_pattern",
"language": "python",
"name": "Context Manager Pattern",
"code": "class MyContextManager:\n def __enter__(self):\n # Setup code\n return self\n def __exit__(self, exc_type, exc_val, exc_tb):\n # Cleanup code\n pass",
"explanation": "Context managers provide a clean way to manage resources like file handles.",
"tags": ["python", "resource management", "context manager"],
"complexity": "intermediate",
"quality_metrics": {
"cyclomatic_complexity": 2,
"documentation_coverage": 0.85
},
"user_rating": 4.5
}
# Store in Qdrant
SQLite Algorithm Database
For maintaining a structured catalog of algorithms with proper versioning.
Database Schema:
-
algorithms
: Basic algorithm information (name, description) -
algorithm_versions
: Different versions of algorithm implementations -
algorithm_categories
: Categories like Sorting, Searching, Graph, etc. -
performance_metrics
: Performance data for different implementations -
improvements
: Tracked improvements between versions -
change_logs
: Detailed logs of changes with rationale and context
Version Diffing:
- Store diffs between algorithm versions
- Track performance improvements across versions
- Document rationale behind changes
Example Query:
-- Find all sorting algorithms with performance metrics
SELECT a.name, a.description, v.version_number, p.time_complexity, p.space_complexity
FROM algorithms a
JOIN algorithm_versions v ON a.id = v.algorithm_id
JOIN performance_metrics p ON v.id = p.version_id
JOIN algorithm_category_mapping m ON a.id = m.algorithm_id
JOIN algorithm_categories c ON m.category_id = c.id
WHERE c.name = 'Sorting'
ORDER BY a.name, v.version_number DESC;
-- Get change logs for a specific algorithm
SELECT v.version_number, c.change_description, c.rationale, c.created_at
FROM algorithm_versions v
JOIN change_logs c ON v.id = c.version_id
WHERE v.algorithm_id = 5
ORDER BY v.version_number;
Knowledge Graph Integration
For representing complex relationships between coding concepts, patterns, and solutions.
Advanced Ontology:
- Algorithm
- DesignPattern
- CodeConcept
- ProblemType
- Solution
- Framework
- Library
- Language
Rich Relation Types:
- IMPLEMENTS (Algorithm → CodeConcept)
- SOLVES (DesignPattern → ProblemType)
- OPTIMIZES (Algorithm → Performance)
- RELATED_TO (Any → Any)
- IMPROVES_UPON (Solution → Solution)
- ALTERNATIVELY_SOLVES (Solution → ProblemType)
- EXTENDS (Pattern → Pattern)
- DEPENDS_ON (Solution → Library)
- COMPATIBLE_WITH (Framework → Language)
Graph Analytics:
- Identify frequently co-occurring patterns
- Discover emerging trends in coding practices
- Map problem domains to solution approaches
Usage Workflows
1. Enhanced Problem-Solving Workflow
When facing a new coding problem:
-
Context Gathering:
- Clearly define the problem and constraints
- Identify performance requirements and environment details
- Document project-specific considerations
-
Memory Querying:
- Break down the problem using sequential thinking
- Query Qdrant for similar solutions:
qdrant-find-memories("efficient way to traverse binary tree")
- Filter results by language, complexity, and quality metrics
- Check algorithm database for relevant algorithms:
SELECT * FROM algorithms WHERE name LIKE '%tree%'
- Explore knowledge graph for related concepts and alternative approaches
-
Solution Application:
- Test and verify solution in REPL
- Document performance characteristics
- Compare against alternatives
-
Feedback Loop:
- Store successful solution back in Qdrant with detailed metadata
- Log performance metrics and usage context
- Update knowledge graph connections
2. Pattern Learning & Storage
When discovering a useful pattern:
-
Automated Documentation:
- Generate initial documentation using AI tools
- Include detailed usage examples
- Document edge cases and limitations
-
Quality Assessment:
- Run linters and static analyzers to ensure code quality
- Calculate and store quality metrics
- Validate against best practices
-
Metadata Enrichment:
- Document the pattern with clear examples
- Add comprehensive metadata (language, complexity, dependencies)
- Apply consistent tagging from controlled vocabulary
-
Knowledge Integration:
- Store in Qdrant with appropriate tags and explanation
- Create knowledge graph connections to related concepts
- Add to SQL database if it's an algorithm implementation
- Suggest automatic connections based on content similarity
3. Project Setup & Boilerplate
When starting a new project:
-
Template Selection:
- Choose from library of project templates
- Customize based on project requirements
- Select language, framework, and testing tools
-
Automated Setup:
- Generate project structure with proper directory layout
- Set up version control with appropriate .gitignore
- Configure linting and code quality tools
- Initialize testing framework
-
Best Practices Integration:
- Query memory system for relevant boilerplate code
- Retrieve best practices for the specific project type
- Use stored documentation templates for initial setup
- Configure CI/CD based on project requirements
Security & Data Integrity
-
Access Controls:
- Role-based access for sensitive code repositories
- Permissions for viewing vs. modifying memories
-
Backup & Recovery:
- Regular backups of Qdrant and SQLite databases
- Version control for knowledge graph
- Recovery procedures for data corruption
-
Sensitive Information:
- Sanitize code examples to remove sensitive data
- Validate code snippets before storage
- Flag and restrict access to sensitive patterns
Monitoring & Analytics
-
Usage Tracking:
- Monitor which patterns are most frequently retrieved
- Track search query patterns to identify knowledge gaps
- Log user ratings and feedback
-
Performance Metrics:
- Monitor database response times
- Track memory usage and scaling requirements
- Optimize queries based on usage patterns
Maintenance Guidelines
- Quality over Quantity: Only store high-quality, well-documented code
- Regular Review: Periodically review and update stored patterns
- Contextual Storage: Include usage context with each stored pattern
- Versioning: Track improvements and versions in SQLite
- Tagging Consistency: Use controlled vocabulary for better retrieval
- Performance Optimization: Regularly optimize database queries
- Feedback Integration: Update patterns based on usage feedback
Getting Started
-
Store your first code memory:
qdrant-store-memory(json.dumps({ "type": "code_pattern", "name": "Python decorator pattern", "code": "def my_decorator(func):\n def wrapper(*args, **kwargs):\n # Do something before\n result = func(*args, **kwargs)\n # Do something after\n return result\n return wrapper", "explanation": "Decorators provide a way to modify functions without changing their code.", "tags": ["python", "decorator", "metaprogramming"], "complexity": "intermediate" }))
-
Retrieve it later:
qdrant-find-memories("python decorator pattern")
Future Enhancements
- Advanced code quality assessment before storage
- Integration with version control systems
- Learning from usage patterns to improve retrieval
- Automated documentation generation
- Custom IDE plugins for seamless access
- Multi-modal storage (code, diagrams, explanations)
- Natural language interface for querying
- Performance benchmark database
- Install script for MCP Servers and DB
相关推荐
Evaluator for marketplace product descriptions, checks for relevancy and keyword stuffing.
I find academic articles and books for research and literature reviews.
Confidential guide on numerology and astrology, based of GG33 Public information
Converts Figma frames into front-end code for various mobile frameworks.
Advanced software engineer GPT that excels through nailing the basics.
Take an adjectivised noun, and create images making it progressively more adjective!
Entdecken Sie die umfassendste und aktuellste Sammlung von MCP-Servern auf dem Markt. Dieses Repository dient als zentraler Hub und bietet einen umfangreichen Katalog von Open-Source- und Proprietary MCP-Servern mit Funktionen, Dokumentationslinks und Mitwirkenden.
Ein einheitliches API-Gateway zur Integration mehrerer Ethercan-ähnlicher Blockchain-Explorer-APIs mit Modellkontextprotokoll (MCP) für AI-Assistenten.
Die All-in-One-Desktop & Docker-AI-Anwendung mit integriertem Lappen, AI-Agenten, No-Code-Agent Builder, MCP-Kompatibilität und vielem mehr.
Reviews
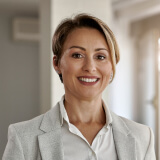
user_fjX4ZzJA
As a dedicated MCP application user, I highly recommend checking out coder_db by angrysky56. This powerful tool has vastly improved my database management tasks, making them more efficient and streamlined. The seamless integration and user-friendly interface allowed me to focus more on coding rather than fiddling with complex database setups. You can find more information at https://github.com/angrysky56/coder_db. Give it a try and see how it can enhance your workflow!